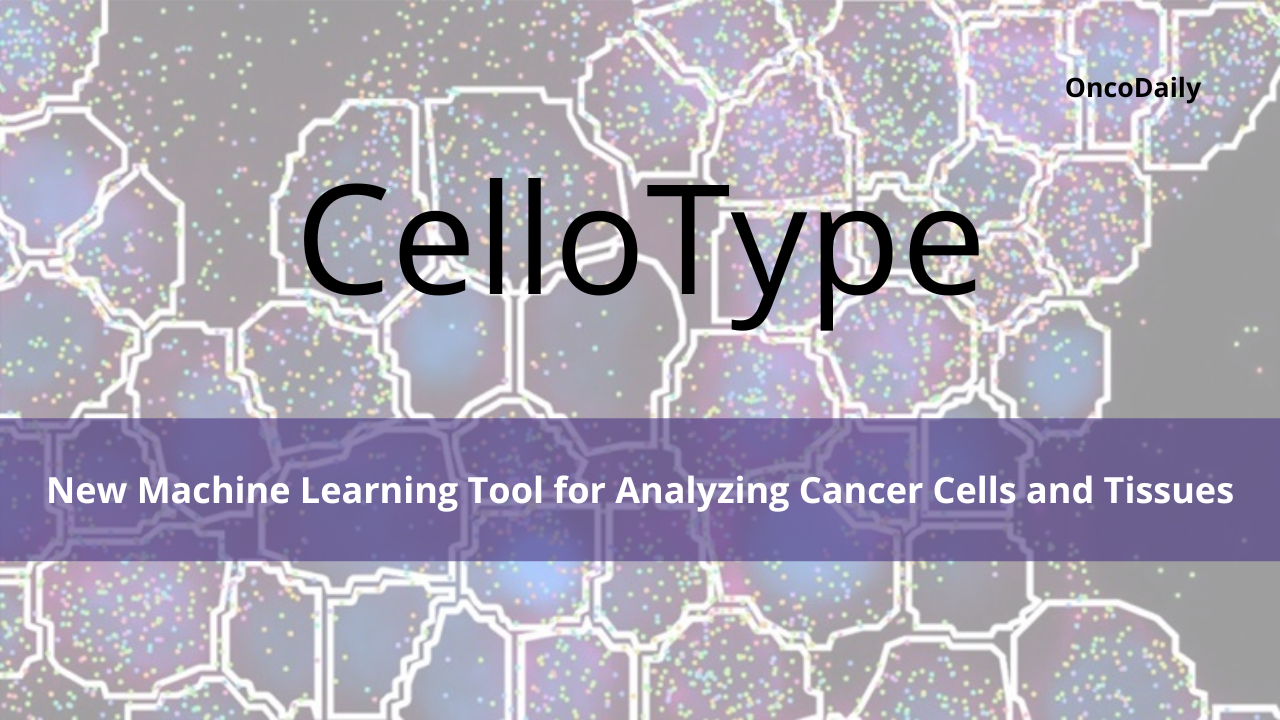
CelloType: A New Machine Learning Tool for Analyzing Cancer Cells and Tissues
NCI-funded researchers have developed a new tool called “CelloType” that uses machine learning to analyze cancer cells and tissues.
This tool provides a straightforward way to study both cellular and non-cellular features in cancer samples.
In a recent study, CelloType proved effective at identifying a wide range of features. It could distinguish non-cellular elements like blood vessels and bone tissue, as well as cells with different shapes, such as immune cells and fat cells, which are often hard to identify.
Corresponding author, Dr. Kai Tan, Professor in the Department of Pediatrics and an investigator in the Center for Childhood Cancer Research of the Children’s Hospital of Philadelphia and the University of Pennsylvania, said:
“Today’s spatial omics technologies rely on accurate cell segmentation. We can apply CelloType to a wide variety of images and classify highly diverse cell types. This information enables us to better understand gene and protein expression and other cancer causing events linked to those specific cells.
We are just beginning to unlock the potential of spatial omics technologies. CelloType advances spatial omics by providing a robust, scalable tool for analyzing complex tissue architectures, thereby expediting discoveries in cellular interactions, tissue function, and disease mechanisms.”
chop.edu
The tool helps researchers label cell types and also allows them to divide and classify these structures. The software is available on GitHub for those interested in using it.
CelloType: a unified model for segmentation and classification of tissue images
Authors: Minxing Pang, Tarun Kanti Roy, Xiaodong Wu, Kai Tan
NCI’s Program Officer, Dr. Miguel Ossandron, added:
“Spatial transcriptomic technologies can generate enormous amount of data (e.g., profiling hundreds of thousands of genes at the single cell level), challenging current methods for analysis. Deep learning tools like CelloType facilitate the annotation of omics data and streamline the way we profile the genes and gene products that drive cancer.
Different from traditional methods, CelloType implements a multi-task learning approach, improving performance and offering a unified framework for detecting, segmenting, and classifying cellular components. These technologies are poised to revolutionize the way we diagnose, treat, and track cancer’s progression.”
About CelloType
CelloType is a deep learning framework tailored for the joint segmentation and classification of diverse biomedical microscopy images, including multiplexed molecular, histological, and bright-field images. Its design leverages advanced neural architectures to process images at multiple scales for precise analysis.
How CelloType Works:
1. Multiscale Feature Extraction:
– The framework begins with the Swin Transformer, which captures image details at various scales, providing a robust set of features for further analysis.
2. Preliminary Detection:
– These features are passed to the DINO object detection module, which identifies individual objects in the image.
– It generates:
– Initial bounding boxes.
– Corresponding class labels for each detected object.
3. Refined Segmentation:
– Outputs from DINO and Swin Transformer are integrated by the MaskDINO module to produce:
– High-resolution segmentation masks for each detected instance.
– Improved bounding box accuracy and classification.
Training Approach
– CelloType optimizes its performance using a comprehensive loss function that evaluates:
– Segmentation accuracy.
– Detection precision.
– Classification reliability.
This joint training process ensures that CelloType effectively handles the complexity of biomedical images, providing detailed and accurate results for both segmentation and classification tasks.
“If you’re using spatial omics technologies, you know how important it is to start with accurate cell segmentation. Check out this new NCI-funded tool, called ‘CelloType,’ which may help automate this task.”
-
Challenging the Status Quo in Colorectal Cancer 2024
December 6-8, 2024
-
ESMO 2024 Congress
September 13-17, 2024
-
ASCO Annual Meeting
May 30 - June 4, 2024
-
Yvonne Award 2024
May 31, 2024
-
OncoThon 2024, Online
Feb. 15, 2024
-
Global Summit on War & Cancer 2023, Online
Dec. 14-16, 2023