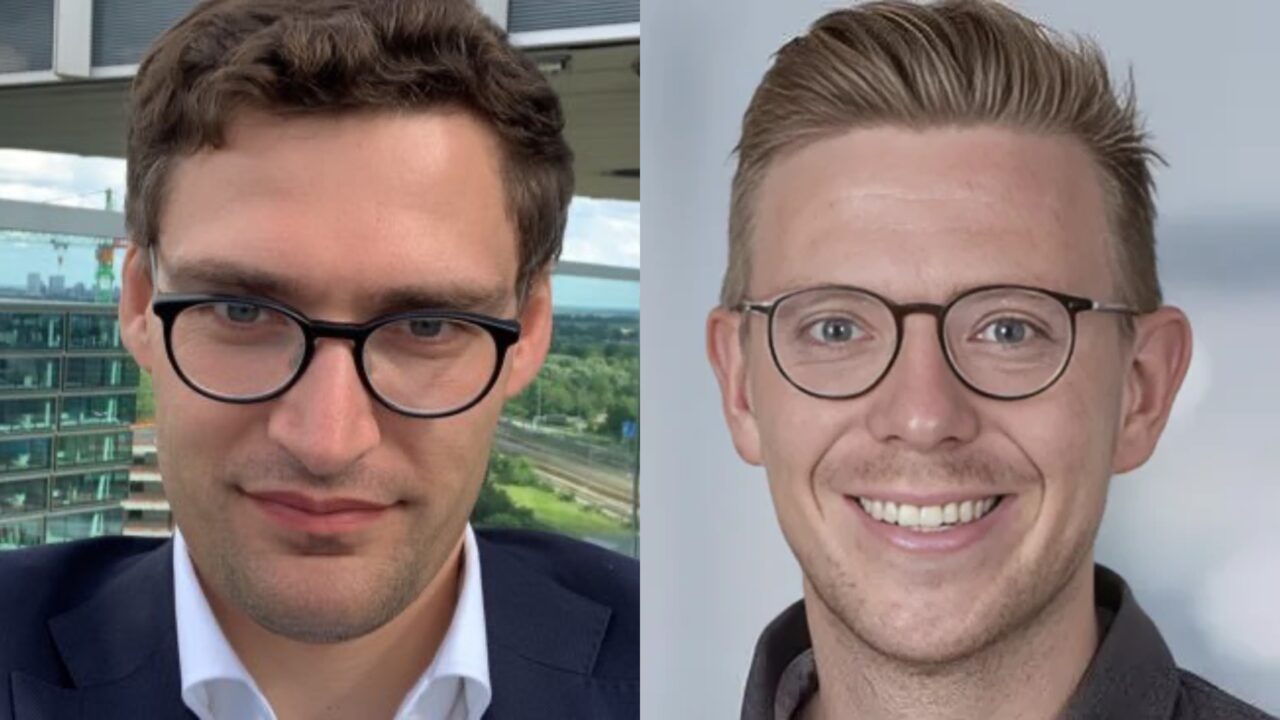
Markus Eckstein: Erdafitinib is just around the corner for metastatic urothelial cancer
Markus Eckstein, Senior Physician/Pathologist and Clinician Scientist at the University Hospital Erlangen, shared a post on X:
“Erdafitinib is just around the corner for metastatic urothelial cancer!
However, FGFR3 alterations are rare (10-15%) in MUC!
In our recently published study in Nature Communications, we thus asked whether we could use AI to prescreen histopathology slides forthe presence of FGFR3 alterations!
Study Design
- Trained on 1222 cases from multiple cohorts
- Achieved AUC=0.82 in cross-validation
- Validated externally on TCGA (AUC=0.82), MIBC II (AUC=0.89), and mUC (AUC=0.82)
Why It Matters
FGFR3 testing often relies on PCR or NGS, which is pricey and time-consuming. This AI model screens slides first, flagging suspicious samples – which potentially could result in fewer molecular tests and faster results in the future (although this has to be proven!).
Performance Highlights
- Near-perfect NPV (0.99–1.00) across cohorts
- Could reduce molecular testing by 36–47% if performance holds true in other data sets.
- Sensitivity nearly 100% for metastatic UC
Critical Note 1: Specificity
Although high sensitivity is great for a pre-screen, specificity is moderate (0.32–0.47). This implies many false positives that still need confirmatory testing.
Critical Note 2: Hotspots and Resolution
- Some FGFR3 mutations (e.g., S249C) are easier to detect.
- Training at 1.0 MPP outperformed 0.5 MPP, suggesting resolution matters.
Model Generalizability
- Performs well across diverse histological subtypes and growth patterns.
- Incorporating NMIBC cases during training unexpectedly boosted accuracy, likely by broadening morphologic representation.
Limitations
- Doesn’t capture FGFR3 fusions well (too few training samples; clear limitation of AI for predicting very rare alterations)
- Some outlier ‘low-expressor’ FGFR3-mutant tumors mimic wild-type morphology and can be missed.
- Additional external validation needed, ideally in a blinded, real-world setting.
Clinical Potential
If validated further, this approach could save time and costs by ruling out most FGFR3 wild-type cases before expensive molecular tests—especially crucial for patients who might benefit from FGFR3-targeted therapies like erdafitinib.
Takeaway
AI pre-screening of routine H&E slides could speed up and reduce costs for FGFR3 testing in bladder cancer. But specificity and fusion detection remain open challenges. Larger trials are the next step to confirm clinical impact.”
Niklas Klümper, Senior Urology Consultant at the University Medical Center Bonn, shared this post, adding:
“Really great paper by Markus Eckstein and Team! Congrats.
AI can guide pre-selection for FGFR3 testing. This has the potential to more broadly assess druggable FGFR alteration in UC!
Especially in settings where FGFR testing is rarely performed..”
Emanuele Crupi, Fellow in Medical Oncology at San Raffaele University-Hospital, shared Markus Eckstein’s post too, adding:
“Another outstanding paper made by an incredible team.
Kudos to Markus Eckstein, Niklas Klümper and team.
A must-read!”
Authors: Pierre-Antoine Bannier et al.
More posts featuring Markus Eckstein, Niklas Klümper and Emanuele Crupi.
-
Challenging the Status Quo in Colorectal Cancer 2024
December 6-8, 2024
-
ESMO 2024 Congress
September 13-17, 2024
-
ASCO Annual Meeting
May 30 - June 4, 2024
-
Yvonne Award 2024
May 31, 2024
-
OncoThon 2024, Online
Feb. 15, 2024
-
Global Summit on War & Cancer 2023, Online
Dec. 14-16, 2023