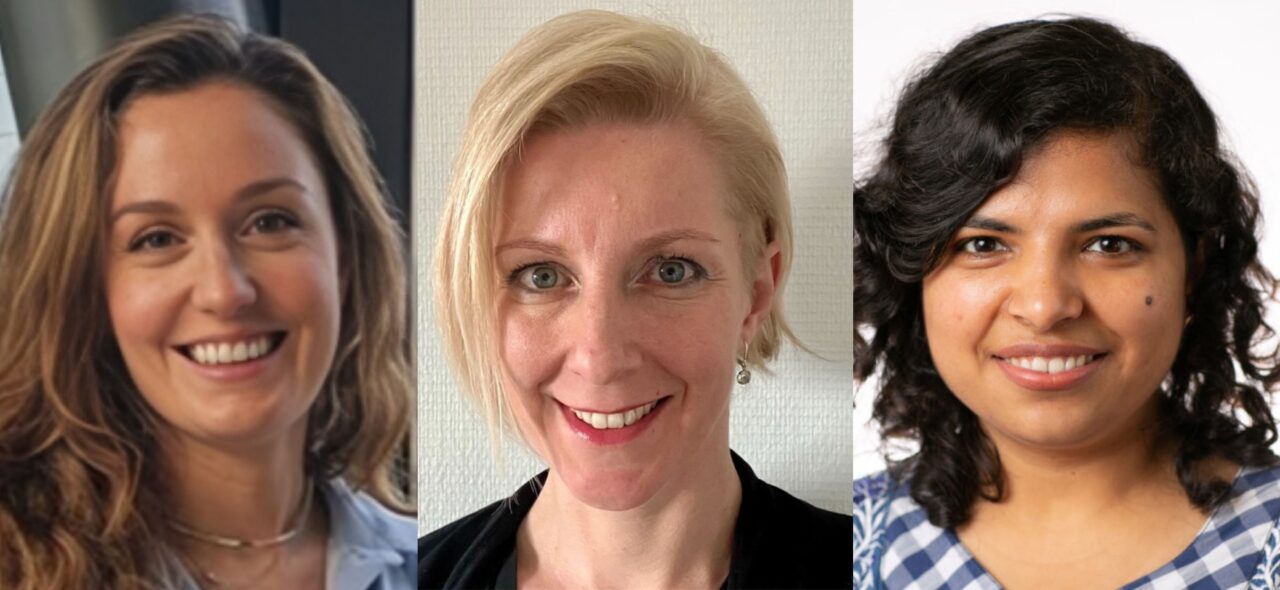
Maria (Masha) Babak: The HECTOR deep learning model holds immense promise in improving personalized treatment strategies
Maria (Masha) Babak shared a post by The Babak Lab on LinkedIn:
“Don’t miss out on this groundbreaking research of Sarah Volinsky, Nanda Horeweg, and Sonali Andani’s article “Prediction of recurrence risk in endometrial cancer with multimodal deep learning” reviewed by The Babak Lab. The HECTOR deep learning model holds immense promise in improving personalized treatment strategies.”
Quoting The Babak Lab’s post:
“Clinical Monday: Revolutionizing Endometrial Cancer Prognosis: HECTOR’s Deep Learning Breakthrough!
We are excited to share our review of the insightful article “Prediction of recurrence risk in endometrial cancer with multimodal deep learning” by Sarah Volinsky, Nanda Horeweg, and Sonali Andani!
The study presents HECTOR (histopathology-based endometrial cancer tailored outcome risk), a cutting-edge deep learning prognostic model for predicting distant recurrence in endometrial cancer (EC). By leveraging hematoxylin and eosin-stained whole-slide images and tumor stage data from 2,072 patients, HECTOR demonstrates superior accuracy compared to the current gold standard. Key results include impressive C-indices and the ability to better predict adjuvant chemotherapy benefits, heralding a new era of personalized treatment for EC patients.
Key Highlights:
- Application of Deep Learning: The research showcases the power of deep learning in predicting EC recurrence risk, highlighting its potential to revolutionize personalized medicine.
- Gating-based Attention and Fusion: Innovative use of three embeddings with gating-based attention and Kronecker product fusion enhances model accuracy.
- Risk Score Definition: Defined risk scores based on predicted probabilities provide a clearer assessment of recurrence risk.
- Quantitative Spatial Analysis: Detailed spatial analysis identifies regions influencing risk scores, offering deeper insights into tumor behavior.
- Impact of Surgical Staging Lymphadenectomy: Findings underline the significance of surgical staging lymphadenectomy in enhancing prognostic accuracy.
- Risk Overestimation: The study acknowledges potential risk overestimation in rare cases, emphasizing cautious interpretation.
- Importance of Data Sharing: Stresses the critical role of data sharing in advancing EC recurrence risk prediction research.
- Related Works: Connects with related deep learning-based cancer survival prediction studies, enriching the research context.
Performance Highlights:
- C-indices: HECTOR achieved C-indices of 0.789, 0.828, and 0.815 in internal and external test sets, surpassing the current gold standard.
- Risk Stratification: Kaplan–Meier analysis revealed distinct outcomes among HECTOR risk groups, with 10-year distant recurrence-free probabilities of 97.0%, 77.7%, and 58.1% for low-, intermediate-, and high-risk groups, respectively.
Why It Matters:
- HECTOR represents a significant leap forward in personalized treatment for endometrial cancer, offering a more accurate, cost-effective method for predicting recurrence risk. This innovation not only enhances clinical decision-making but also underscores the transformative potential of deep learning in healthcare.
Read the full article to explore how HECTOR could shape the future of endometrial cancer treatment and prognosis.”
Additional information.
Source: Maria (Masha) Babak/LinkedIn and The Babak Lab/LinkedIn
Dr. Maria (Masha) Babak is the Head of The Babak Lab and an Assistant Professor at the City University of Hong Kong. She earned her Ph.D. in bioinorganic chemistry from the University of Vienna in 2014. From 2015 to 2020, Dr. Babak was a postdoctoral research fellow at the National University of Singapore under the mentorship of Prof. Wee Han Ang, where she developed a strong passion for drug discovery and drug target identification. In November 2020, she joined the City University of Hong Kong as an assistant professor. Dr. Babak received the Graeme Hanson-AsBIC Early Career Award in 2022.
Her research interests are at the intersection of chemistry, biology, and medicine, with a focus on the discovery and preclinical development of anticancer drugs for resistant and aggressive cancers with limited treatment options, such as malignant pleural mesothelioma and brain metastases..
-
ESMO 2024 Congress
September 13-17, 2024
-
ASCO Annual Meeting
May 30 - June 4, 2024
-
Yvonne Award 2024
May 31, 2024
-
OncoThon 2024, Online
Feb. 15, 2024
-
Global Summit on War & Cancer 2023, Online
Dec. 14-16, 2023