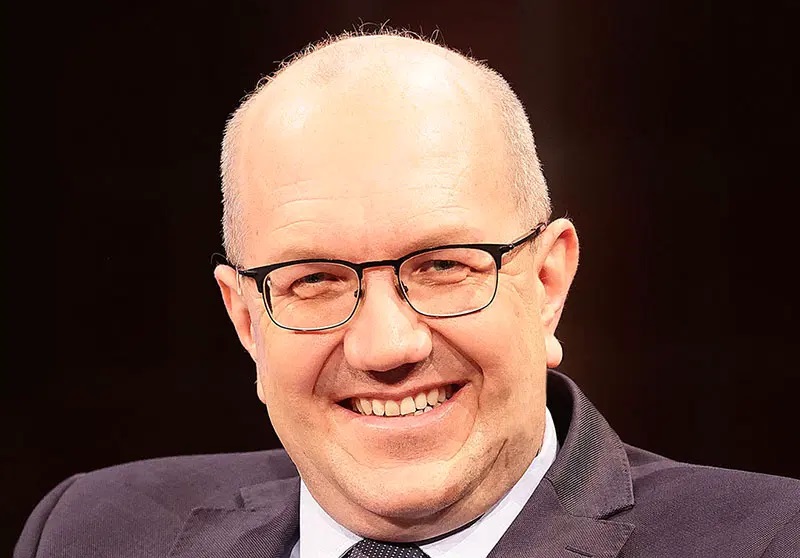
Sebastian Schmidt: Overdiagnosis in Lung Cancer (Part 1) – is it real? And is it a problem?
Sebastian Schmidt, the Head of Strategy and Medical Affairs Computed Tomography at Siemens Healthineers, shared on LinkedIn:
“Overdiagnosis in Lung Cancer (1/4) – is it real? And is it a problem?
Overdiagnosis in lung cancer screening is a topic that is repeatedly discussed, time to put some findings in a context.
It’s important to correctly define Overdiagnosis: It’s the (correct) diagnosis of a disease that, without diagnosis would not have lead to symptoms or the death of the patient. It has nothing (!) to do with false positives.
Overdiagnosis is inherent to diagnostics – if you make a diagnosis, there is risk of overdiagnosis. The most exaggerated example is a person who gets a correct diagnosis from you and is hit over by a bus when leaving your office. This shows one point: The quicker the natural progress of a disease, the lower the risk of overdiagnosis (lung vs. prostate CA).
How to assess overdiagnosis rates from screening study data?
If you plot incidence (rate of diagnosis) of the two groups, screened and unscreened, what you see is a left-shift of the screened group showing earlier diagnosis, which is the purpose of screening, and an upward shift, that is (more or less) overdiagnosis, as these diagnoses did not show up in the control group (likely neither through symptoms nor death).
The challenge: As long as both curves grow, it’s impossible to differentiate left and upward. It just looks the same. This would require very long follow-up times.
Statisticians use “imputation” (which, for me, seems to “educated guessing” in academic language). But let’s look at one of the examples with long f-up.
The charts below from three studies illustrate it:
1. Hitachi study in Japan (DOI: 10.1093/jjco/hyy185). Here you see the two incidence curves for CT screening vs. X-Ray (XP). It looks like a mix of left-shift (earlier diagnosis) and upward (overdiagnosis), illustrates the concept.
2. NLST study (DOI: doi:10.1056/NEJMoa1102873). This what that Patz et al used to calculate the 18.5% overdiagnosis that is often cited (DOI: 10.1001/jamainternmed.2013.12738). Here it seems like if both curves run flat and parallel at the end of the period.
3. Again NLST, same population, longer follow-up (DOI: 10.1016/j.jtho.2019.05.044). Here we see “overdiagnosis” disappearing with longer follow-up – almost the same rate of lung cancer, just diagnosed later. We also see that the effect of earlier diagnosis disappears, which comes from the study design with 3 annual CT rounds in the beginning and then just follow-up. This contributes to the strong underestimation of the screening benefit by the NLST, which I commented on in an earlier post.
Another point: Overdiagnosis is almost completely attributed to slow-growing carcinomas like BAC (Bronchoalveolar carcinoma), taking long to cause symptoms.
Conclusion: At least in Western countries, there are little signs that overdiagnosis in lung cancer is a bigger issue. Still doctors doing screening should be aware of this risk. In Asia, things may be different – I will follow-up on this and more aspects in later posts, stay tuned.”
Source: Sebastian Schmidt / LinkedIn
-
ESMO 2024 Congress
September 13-17, 2024
-
ASCO Annual Meeting
May 30 - June 4, 2024
-
Yvonne Award 2024
May 31, 2024
-
OncoThon 2024, Online
Feb. 15, 2024
-
Global Summit on War & Cancer 2023, Online
Dec. 14-16, 2023