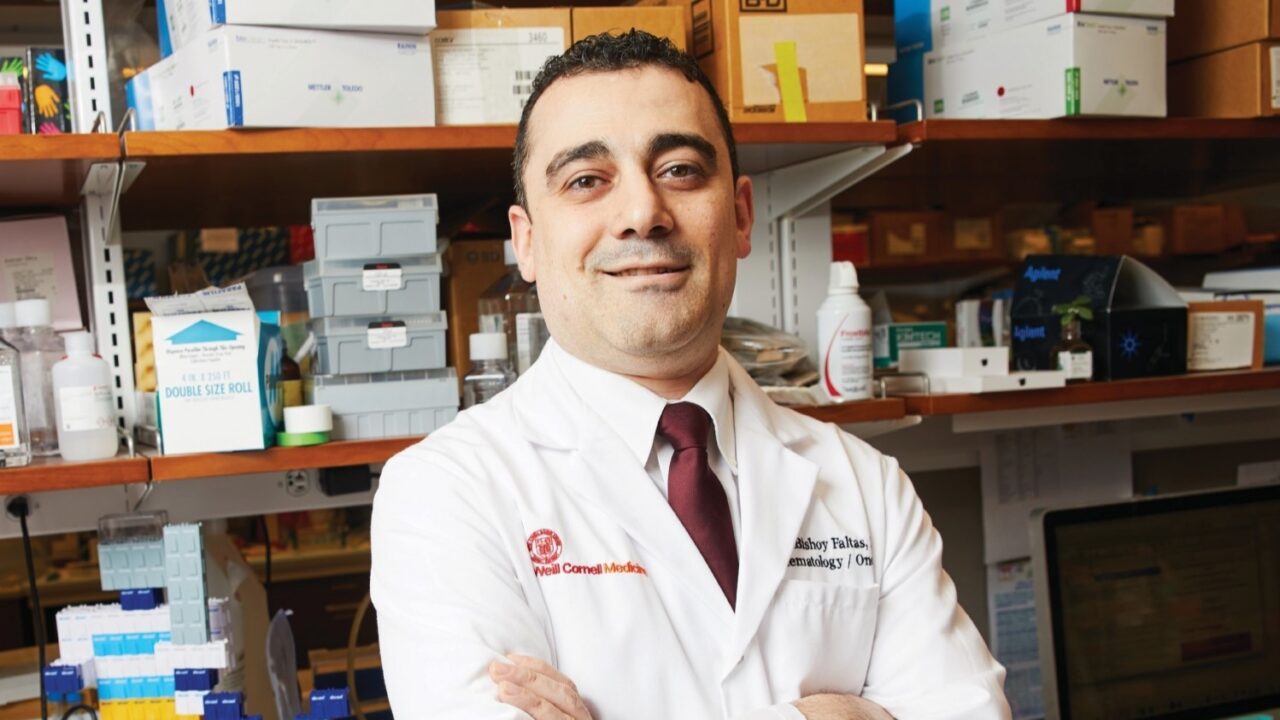
Predicting response to neoadjuvant chemotherapy in MIBC via interpretable multimodal deep learning
Bishoy M. Faltas, Chief Research Officer at the Englander Institute for Precision Medicine and an Associate Professor of Medicine and Cell and Developmental Biology at Weill Cornell Medicine, posted on X about recent paper he and his colleagues co-authored:
“Excited to share our new paper in npj Digital Medicine. We worked with Fei Wang to develop a cutting-edge AI model that predicts response to neoadjuvant chemotherapy in patients with muscle-invasive bladder cancer in the S1314 randomized clinical trial.
This is a team science collaboration spearheaded by Zilong Bai, Mohamed Osman and with contributions from the S1314 clinical trial team,SWOG Cancer Research Network, David McConkey, Thomas Flaig, Dan Theodorescu, Sia Daneshmand, Joshua Meeks, Woonyoung Choi and my Weill Cornell Medicine, Englander Institute for Precision Medicine colleagues.
A standard-of-care treatment for muscle-invasive bladder cancer is neoadjuvant chemotherapy followed by surgery to remove the bladder. ~35% of patients experience a complete response to neoadjuvant chemotherapy meaning that there is no cancer left after chemotherapy.
If we could predict which patients are going to have a complete response, these patients can potentially avoid the surgery and preserve their bladders. This is currently being tested in clinical trials but our ability to select these patients is currently very limited.
To solve this problem, we developed a state-of-the-art deep learning model to fuse three neural networks trained on:
- Pathology whole-slide images
- Spatial cell data
- Gene expression
This multimodal integration approach significantly outperformed any single data type alone and predicts response to chemotherapy with a high accuracy.
One of the most important concerns about AI is the black box problem. We don’t always know how the AI models make their predictions. This poses a significant challenge, especially in healthcare decision-making.
We unlocked this “black box” through robust interpretability methods. Our model doesn’t just predict – it explains WHY it made those predictions. We used SHapley Additive exPlanations (SHAP), values that are derived from a game theory approach that measures each player’s contribution to the outcome to help us interpret our model’s predictions.
SHAP analysis showed that the gene expression branch outweighed the whole slide image features in determining the complete response. It also identified that specific biologically significant genes (TP63, CCL5, DCN) drive these predictions.
Our model revealed tumor architectural patterns associated with treatment response, such as higher tumor-stromal ratio. This generated new hypotheses of histopathological determinants of response, including cell-type spatial patterns within the tumor microenvironment that traditional analyses might overlook. In other words, the model generated new hypotheses that we are testing!
In summary, we built a multi-modal framework that integrates clinical and genetic data to predict response to therapy in patients enrolled in Clinical Trials. We are now working on adding additional data streams to this framework to integrate HandE images, genomic and transcriptomic data , ctDNA, and CT scans. This is one key piece of the integrated ecosystem that our team is building Weill Cornell Medicine where AI meets Oncology.
This is only the first piece of the puzzle. Stay tuned in the next few weeks more!”
Predicting response to neoadjuvant chemotherapy in muscle-invasive bladder cancer via interpretable multimodal deep learning
Authors: Zilong Bai, Bishoy M. Faltas, et al.
More posts featuring Bishoy M. Faltas.
-
Challenging the Status Quo in Colorectal Cancer 2024
December 6-8, 2024
-
ESMO 2024 Congress
September 13-17, 2024
-
ASCO Annual Meeting
May 30 - June 4, 2024
-
Yvonne Award 2024
May 31, 2024
-
OncoThon 2024, Online
Feb. 15, 2024
-
Global Summit on War & Cancer 2023, Online
Dec. 14-16, 2023