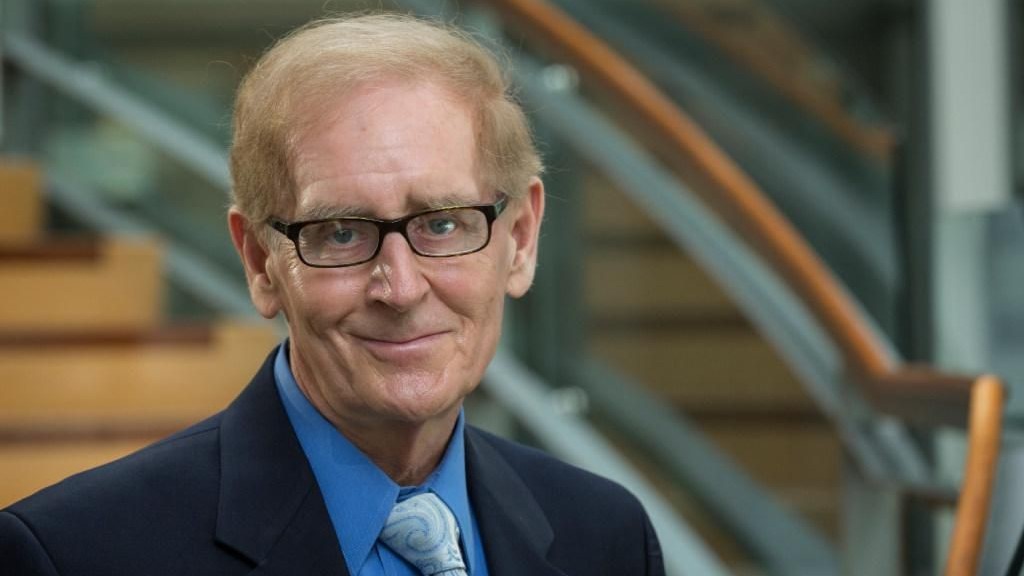
Gary Lyman: Balancing innovation and human insight – navigating the promise and limitations of AI in cancer research
Gary Lyman, Adjunct Professor of Medicine at Duke University School of Medicine, shared a post on X:
“AI is transforming many aspects of medicine. However, AI has notable limitations when applied to complex and deeply human tasks like understanding patient needs and interpreting medical data.”
Read the article from the Gary Lyman research group, Public Health Sciences Division
Artificial Intelligence (AI) is making significant strides in medicine, particularly in cancer research, where accurate predictions are vital for effective treatment. While AI enhances clinical decision-making and patient outcomes, it also faces considerable limitations, especially in understanding human emotions and interpreting complex medical data.
Drs. Gary Lyman and Nicole Kuderer explore these issues in a series of articles published in Cancer Investigation, emphasizing the need for human oversight in healthcare.
Defining Intelligence
Lyman and Kuderer distinguish between human intelligence and machine learning (ML). Human intelligence involves data processing, perception, creativity, and emotional awareness—qualities that AI currently lacks. While AI can analyze vast datasets, it does not possess the subjective experiences that inform human cognition.
AI’s Role in Cancer Research
AI’s impact in cancer research is notable. Machine learning enables the identification of complex patterns in data, enhancing predictions related to diagnostics and treatment planning. Traditional ML relies on labeled data (supervised learning), while unsupervised learning uncovers hidden patterns in unlabeled datasets. Advanced deep learning techniques further improve AI’s ability to manage extensive medical data.
Clinical Prediction Models
In their second article, Lyman and Kuderer discuss AI’s role in developing clinical prediction models that forecast patient outcomes and guide treatment decisions. These models analyze various factors, such as tumor type and genetics, but require high-quality data and rigorous validation to ensure reliability across diverse populations.
Challenges of Bias and Transparency
Bias remains a critical concern in AI healthcare modeling. If training data are skewed, predictions may be inaccurate for broader populations. The “black box” nature of many AI systems complicates understanding their decision-making processes. Additionally, many studies do not adhere to established reporting standards like TRIPOD, hindering replication and validation efforts.
Philosophical Limitations of AI
Lyman and Kuderer also address the philosophical limitations of AI, referencing Gödel’s Incompleteness Theorem and Turing’s Halting Problem to argue that certain truths remain beyond algorithmic reach. These concepts suggest that human cognition’s ability to grasp abstract ideas cannot be fully replicated by AI.
Conclusion
While AI has transformative potential in cancer research by enabling rapid data analysis and supporting clinical decisions, its limitations—including bias, lack of transparency, and inability to replicate human intuition—highlight the importance of integrating human expertise with AI capabilities. Future advancements should focus on improving the ethical application and transparency of AI models in medicine to ensure they effectively enhance cancer care for patients and society.
For more posts like this, visit oncodaily.com.
-
Challenging the Status Quo in Colorectal Cancer 2024
December 6-8, 2024
-
ESMO 2024 Congress
September 13-17, 2024
-
ASCO Annual Meeting
May 30 - June 4, 2024
-
Yvonne Award 2024
May 31, 2024
-
OncoThon 2024, Online
Feb. 15, 2024
-
Global Summit on War & Cancer 2023, Online
Dec. 14-16, 2023