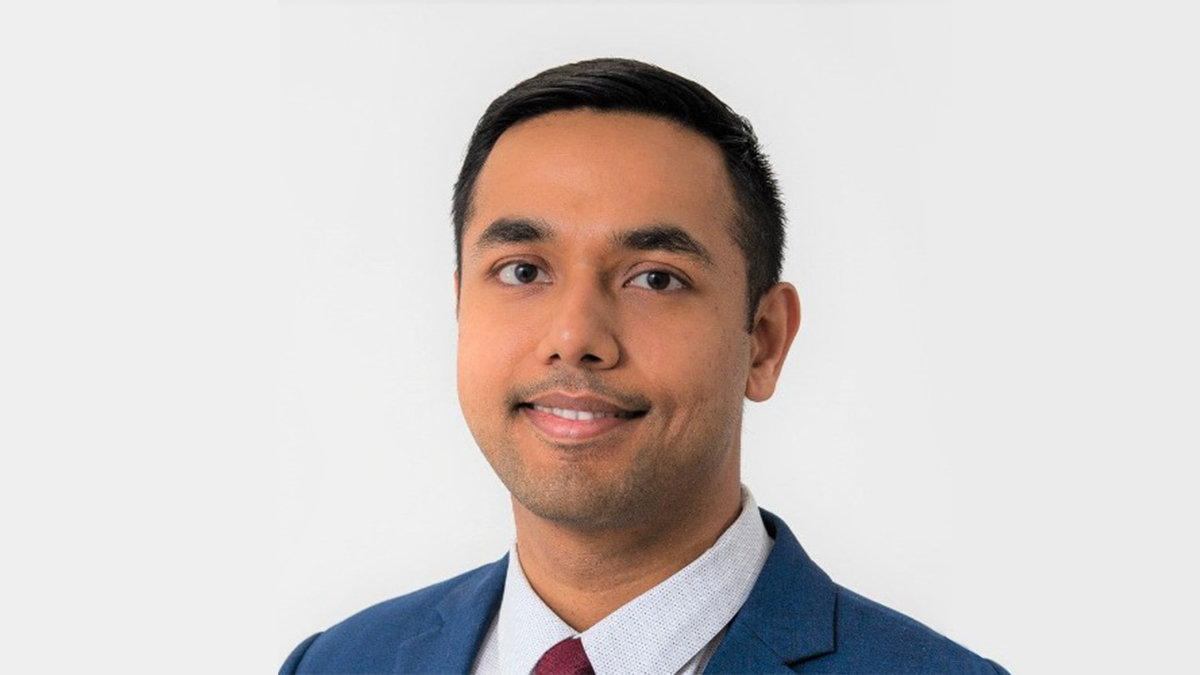
Aakash Desai: Can machine learning outperform PD-L1 as a predictive biomarker for ICI therapy in advanced NSCLC?
Aakash Desai, Assistant Professor at UAB O’Neal Comprehensive Cancer Center, shared on LinkedIn:
“Can machine learning outperform PD-L1 as a predictive biomarker for immune checkpoint inhibitor (ICI) therapy in advanced NSCLC?
A new study by Ravi B. Parikh and colleagues in American Society of Clinical Oncology (ASCO) journal JCO CCI leveraged real-world data from 38,048 patients with advanced NSCLC to train machine learning (ML) algorithms to predict 12-month progression-free survival (PFS) and overall survival (OS) for patients receiving first-line therapy, including ICI monotherapy.
Key Findings:
• ML algorithms achieved an AUC of 0.701 for 12-month PFS and 0.718 for 12-month OS.
• Patients classified as low-risk (LR) by the ML model had significantly better outcomes:
Lower 12-month disease progression: HR 0.47 (P < .001).
Lower 12-month mortality: HR 0.31 (P < .0001).
Key Conclusion: The ML algorithm outperformed PD-L1 alone in predicting response to ICI therapies, demonstrating its potential to guide more precise clinical decision-making in oncology.
As we move toward more personalized cancer care, ML offers a robust complement to existing biomarkers.”
-
ESMO 2024 Congress
September 13-17, 2024
-
ASCO Annual Meeting
May 30 - June 4, 2024
-
Yvonne Award 2024
May 31, 2024
-
OncoThon 2024, Online
Feb. 15, 2024
-
Global Summit on War & Cancer 2023, Online
Dec. 14-16, 2023