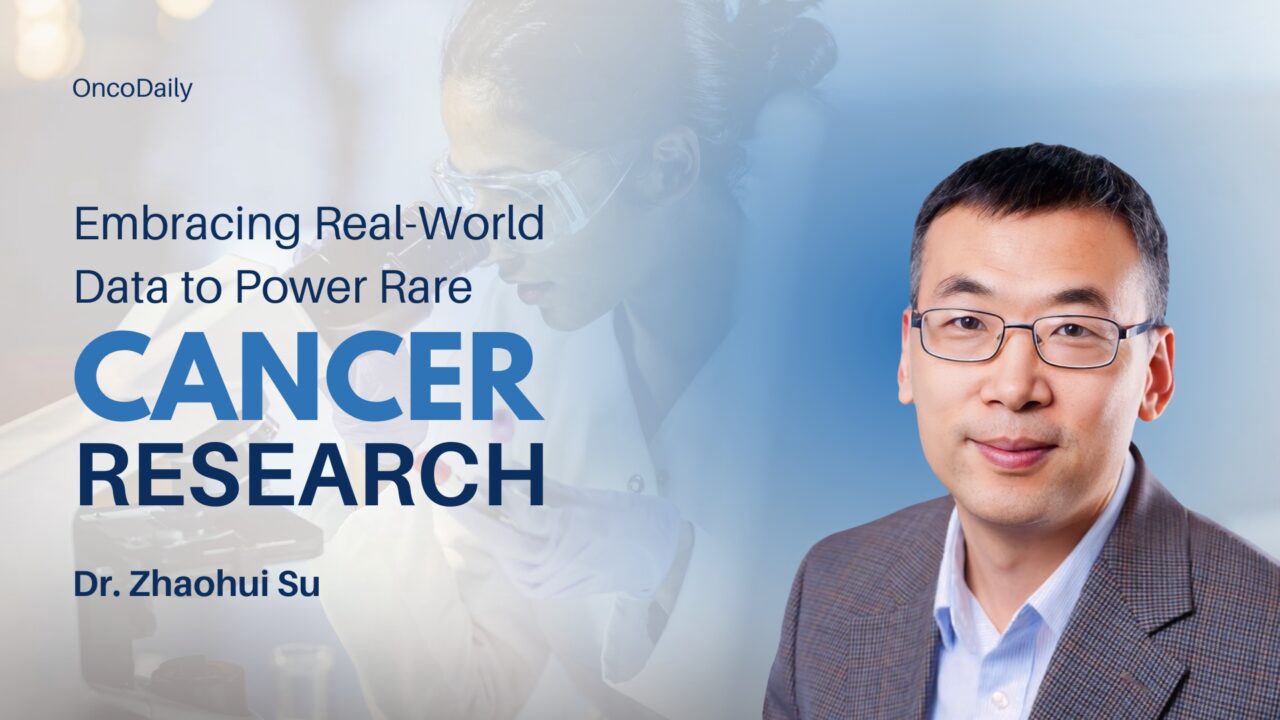
Zhaohui Su: Embracing Real-World Data to Power Rare Cancer Research
“The study of cancer has evolved over the last two decades, driven primarily by genomic profiling and molecular diagnostics, which have exponentially expanded our understanding of the disease. Rather than the overly simplified definition of a ‘disease in which some of the body’s cells grow uncontrollably and spread to other parts of the body’, the oncology community and researchers now look at it as a collection of rare, biomarker-defined subtypes. Our ability to comprehend cancer at the genetic level now drives the vast majority of clinical research and drug development.
This evolved thinking is especially prudent in the case of rare cancers, which now account for up to 25% of all. The identification of actionable biomarkers within these tumors has enabled the development of targeted therapies that have significantly improved overall survival rates – by more than one year compared to non-targeted therapies, according to one study.
While our knowledge of this disease has transformed the treatment landscape, it also has had the unintended consequence of complicating the study of rare cancers, especially in the context of precision medicine and biomarker-driven oncology. Generally speaking, finding patients with specific biomarkers to participate in clinical trials can be both challenging and time-consuming due to the potentially small size of these patient populations, even for more common cancers.
For rare cancers, however, this challenge is magnified, as researchers need to draw from a much smaller pool of patients. Small sample sizes may reduce the generalizability of findings and the statistical power of studies, making it difficult to draw reliable conclusions.
Therefore, it is critical for researchers to design studies with the appropriate statistical power, regardless of the incidence of a particular cancer. If the study is underpowered, it may not detect significant associations or a true effect. An overpowered study may unnecessarily increase the cost and duration of the study. It is essential to determine the appropriate statistical power or precision for estimating the outcomes of interest.
There are several options researchers can explore to help increase the statistical power of a study:
- Large data source(s). Identifying and leveraging a large data source or linking multiple data sources to increase the sample size can help adequately power a study.
- Duration of follow-up. By extending follow-up periods, researchers may be able to gather more data points, increasing the likelihood of detecting significant associations.
- Surrogate endpoints. Selecting appropriate surrogate endpoints, such as pathologic complete response, serve as early indicators of long-term outcomes; from 2006-2022, 71% of oncology drug approvals (non-hematologic solid tumors) were based on surrogate endpoints.
- Select a study design and statistical methods that maximize power. Choose study design and analysis methods that are tailored for small sample sizes, such as a within-subjects design (also known as a repeated measures design), a research design method that assigns participants to treatment groups, exact tests and meta-analyses.
Missing data can reduce statistical power and lead to biased results. To mitigate this, it is critical to assess data completeness and fitness for purpose, particularly in cancers where sample sizes may be limited. Employing machine learning, natural language processing and expert chart abstraction can significantly enhance data extraction and completeness. Data representativeness should be evaluated, and sensitivity analyses are highly recommended.
As the quest for reliable data intensifies, the oncology community is beginning to accumulate a myriad of rich data sets that have the potential to expedite the drug development cycle, particularly through the use of real-world data (RWD) and real-world evidence (RWE).
According to the U.S. Food and Drug Administration (FDA), RWD provides invaluable insights into the effectiveness, safety and value of medical treatments and interventions. In fact, between January 2019 and June 2021, 85% of FDA-approved applications for new drugs had incorporated RWE in some form, and more than half of those approvals noted that the RWE influenced the FDA’s final decision.
There is no debate regarding the importance of clinical research in evaluating the efficacy and safety of an investigational medicine. However, conducting research in rare cancers in a controlled setting may not be generalizable to all patients.123
With small patient populations, RWD may provide important evidence that complements clinical findings and could help fill knowledge gaps related to how a medication is used in real-world medical settings. RWE provides researchers with comprehensive, pragmatic insights into treatment outcomes, and could identify areas for improvement in healthcare, better inform regulatory decisions and policy changes and enable better long-term patient-centered care.
In 2023, Ontada was awarded a contract with the FDA to initiate a real-world study exploring the natural history of patients across 10 rare cancers in U.S. community cancer practices using RWD from the company’s iKnowMed electronic health records (EHR) system and comparing results from approximately 2.4 million patient records with other data sources like national cancer registries. The goal of this research is to facilitate a greater understanding of patient care in the community setting for rare cancers to help inform clinical trial design to better study possible treatments.
RWD is essential to providing key insights into how drugs perform in clinical practice and play an important role in driving clinical research in oncology, particularly for rare cancers where numerous barriers to research exist. I strongly believe in the power of RWD to supplement clinical data which is key to expediting regulatory approvals of effective and safe treatments.”
written by Zhaohui Su
Vice President of Biostatistics at Ontada
Zhaohui Su is the Vice President of Biostatistics at Ontada and a scientific leader with experience applying statistical, epidemiological, and machine learning methods to real-world studies. His expertise includes oncology study design, data organization, epidemiological methods, predictive modeling, and statistical analysis for categorical, continuous, and survival data. Previously, Dr. Su served as Vice President of Real-World Evidence Analytics at OM1, Inc., Senior Director of Biostatistics at Quintiles, and Head of the Optimization of Antiretroviral Therapy Section at the Harvard School of Public Health (HSPH).
1 Kostis JB, Dobrzynski JM. Limitations of randomized clinical trials. Am J Cardiol. 2020;129:109-115. doi: 10.1016/j.amjcard.2020.05.011
2 Frieden T. Why the ‘gold standard’ of medical research is no longer enough. STAT. August 2, 2017. Accessed January 10, 2024.
3 Clay RA. More than one way to measure. Monitor on Psychology. 2010;41(8):52.
-
Challenging the Status Quo in Colorectal Cancer 2024
December 6-8, 2024
-
ESMO 2024 Congress
September 13-17, 2024
-
ASCO Annual Meeting
May 30 - June 4, 2024
-
Yvonne Award 2024
May 31, 2024
-
OncoThon 2024, Online
Feb. 15, 2024
-
Global Summit on War & Cancer 2023, Online
Dec. 14-16, 2023