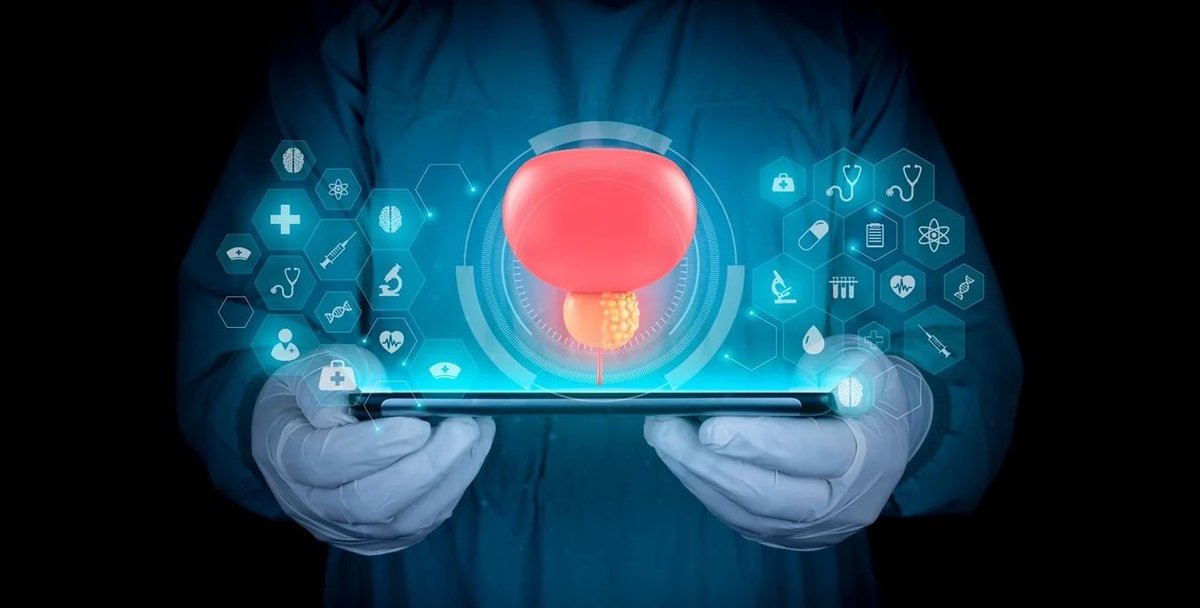
Shahrin Ahmed: The use of machine learning for the diagnosis of prostate cancer
Shahrin Ahmed, Medical Specialist at the Canadian Cancer Society, shared a post on X:
“The use of machine learning for the diagnosis of prostate cancer – Prostate cancer is the most common cancer among men worldwide.
Multiparametric magnetic resonance imaging (MRI) is frequently employed in the diagnosis of csPCa. The prostate imaging reporting and data system (PI-RADS) is utilized in a standardized reporting and interpretation approach, which requires a high level of expertise.
Using DL, csPCa can be detected by training a model on specific regions of interest which are informed by MRI scans. Annotating lesions during the model development and retraining and re-evaluation stages after clinical implementation, a radiologist or pathologist is required in these machine-learning approaches.
The implementation of these approaches is costly and restricts the size of the data set. The present study aimed to build a DL model that can predict the presence of csPCa without requiring prior information on the tumor’s location.
Patient-level labels were used to clarify whether csPCa was present or absent and the model’s predictions were compared with those of radiologists. Between January 2017 and December 2019, data was collected on patients who had no known csPCa and underwent an MRI scan.
The training of a convolutional neural network based on T1-weighted contrast-enhanced images, T2-weighted images, apparent diffusion coefficient maps, and diffusion-weighted images involved using T1-weighted contrast-enhanced images, T2-weighted images, and diffusion-weighted images.
The reference standard was established through pathologic diagnosis. The evaluation included four models: image-only, radiologists, image plus radiologist, and image plus clinical plus radiologist.
Patients with PI-RADS 1 or 2 lesions who did not undergo biopsy accounted for a significant fraction of negative cases when it comes to using Grad-CAM for tumor localization. Several cases were deemed to be false-negative.
By utilizing MRI and a DL model, the current study was able to predict the presence of csPCa. For both internal and external test sets, there were no statistically significant differences between the model’s performance and that of experienced radiologists.
It is clear from these findings that the DL model created in the current study has potential. Radiologists can be assisted in identifying CSPCa and performing lesion biopsy through a program, which has the potential to greatly enhance the diagnosis of prostate cancer.
A significant limitation of the current study is its single-site and retrospective nature.
To enhance its predictive accuracy, the DL model was restricted to radiologists who had expertise in prostate MRI and excluded trainees and general radiologists.”
Source: Shahrin Ahmed/X
-
Challenging the Status Quo in Colorectal Cancer 2024
December 6-8, 2024
-
ESMO 2024 Congress
September 13-17, 2024
-
ASCO Annual Meeting
May 30 - June 4, 2024
-
Yvonne Award 2024
May 31, 2024
-
OncoThon 2024, Online
Feb. 15, 2024
-
Global Summit on War & Cancer 2023, Online
Dec. 14-16, 2023