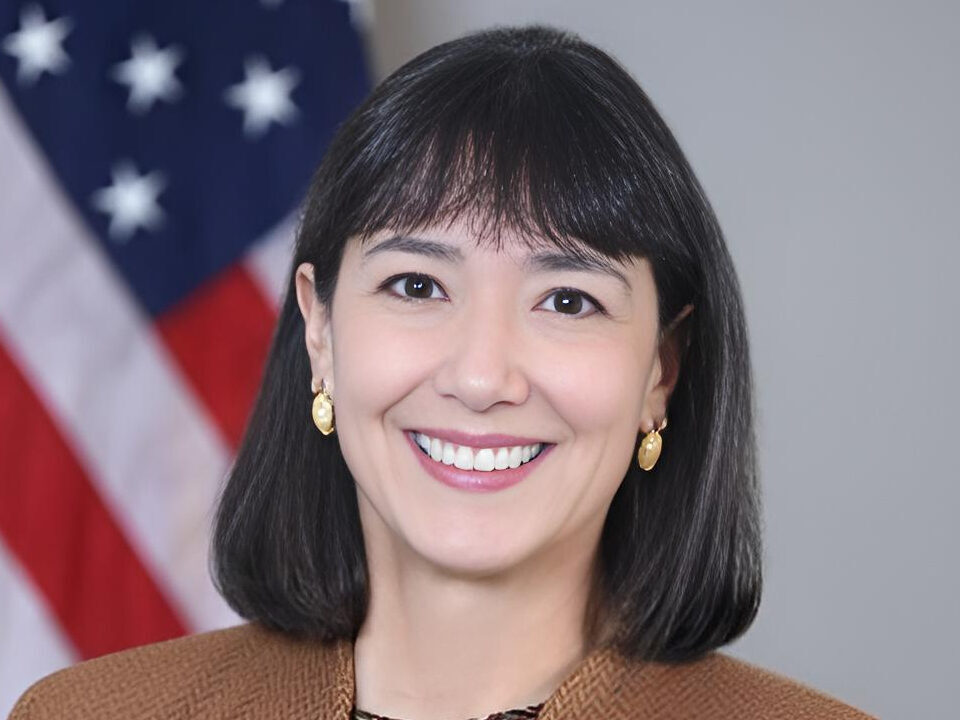
Monica Bertagnolli: AI Tool Has Promise for Matching Cancer Drugs to Patients
Monica Bertagnolli shared a post on LinkedIn:
”AI Tool Using Single-Cell Data Has Promise for Optimally Matching Cancer Drugs to Patients.
Precision oncology, in which doctors choose cancer treatment options based on the underlying molecular or genetic signature of individual tumors, has come a long way. The Food and Drug Administration has approved a growing number of tests that look for specific genetic changes that drive cancer growth to match patients to targeted treatments. The NCI-MATCH trial, supported by the National Cancer Institute, in which participants with advanced or rare cancer had their tumors sequenced in search of genetic changes that matched them to a treatment, has also suggested benefits for guiding treatment through genetic sequencing. But there remains a need to better predict treatment responses for people with cancer.
A promising approach is to analyze a tumor’s RNA in addition to its DNA. The idea is to not only better understand underlying genetic changes, but also learn how those changes impact gene activity as measured by RNA sequencing data. A recent study introduces an artificial intelligence (AI)-driven tool, dubbed PERCEPTION (PERsonalized single-Cell Expression-based Planning for Treatments In ONcology), developed by an NIH-led team to do just this.1 This proof-of-concept study, published in Nature Cancer, shows that it’s possible to fine-tune predictions of a patient’s treatment responses from bulk RNA data by zeroing in on what’s happening inside single cells.
One of the challenges in relying on bulk data from tumor samples is they typically include mixtures of like cells known as clones. Because different clones may respond differently to specific drugs, averaging what’s happening in cells across a particular patient’s tumor may not provide a clear picture of how that cancer will respond to a drug. Being able to capture gene activity patterns all the way down to the single-cell level might be a better way to target clones with specific alterations and therefore see better drug responses, but so far, single-cell gene expression data haven’t been widely available.
To explore the potential of single-cell RNA data, a team led by Eytan Ruppin and Alejandro Schäffer at NCI’s Cancer Data Science Laboratory at the NIH Clinical Center in Bethesda and Sanju Sinha, now at Sanford Burnham Prebys in San Diego, used a technique called transfer learning to train an AI model to predict drug responses. They first used existing bulk RNA sequencing data and then fine-tuned those models using single-cell RNA sequencing data from cell lines and large-scale drug screens. All told, they built AI models for 44 drugs approved by the FDA.
They found that PERCEPTION predicts the success of targeted treatments against cell lines with an accuracy reflected by an AUC score of about 0.8. AUC measures how well a model can distinguish between drug-sensitive and drug-resistant cell lines, with 0.5 being no better than a random guess and 1.0 being perfect accuracy. While there’s room for improvement, the findings show that PERCEPTION works better than earlier methods. The results also extended to single drugs and combination treatments in cultured cells and in cells isolated from patient tumors.
But would the tool accurately predict responses to treatments for patients? To find out, the researchers used their models to predict treatment responses based on clinical trial data for 41 patients with multiple myeloma treated with a combination of four drugs and 33 patients with breast cancer treated with a combination of two drugs. Their findings showed that the model could successfully predict treatment responses in patients, again with an AUC score of about 0.8.
Interestingly, their research shows that having just one clone in a tumor that is resistant to a particular drug is enough to thwart a response to that drug. As a result, the clone with the worst response in a tumor will best explain a person’s overall treatment response. Further study revealed that the model could also predict the development of resistance to treatment in published data from 24 people treated with targeted therapies for non-small cell lung cancer.
The researchers note that the accuracy of their technique will only improve as single-cell RNA sequencing data becomes more widely available for more patients with additional cancer types. To aid in this endeavor, they’ve developed a research website and guide to enable other researchers to use PERCEPTION to build AI models that predict treatment responses. Their hope is, as these findings suggest, that single-cell RNA sequencing data could one day help doctors more precisely match patients to their optimal cancer treatments.
Reference:
[1] Sinha S, et al. PERCEPTION predicts patient response and resistance to treatment using single-cell transcriptomics of their tumors. Nature Cancer. DOI 10.1038/s43018-024-00756-7 (2024).
NIH Support: National Cancer Institute.”
Source: Monica Bertagnolli/LinkedIn
Monica Bertagnolli is the Director of the National Institute of Health (NIH), USA. She previously served as the Richard E. Wilson Professor of Surgery in the field of surgical oncology at Harvard Medical School, a surgeon at Brigham and Women’s Hospital, and a member of the Gastrointestinal Cancer Treatment and Sarcoma Centers at Dana-Farber Cancer Institute. Dr. Bertagnolli is the Founding Chair of the minimal Common Oncology Data Elements (mCODE) executive committee and has held multiple positions nationally, including being Past President and Chair of the Board of Directors of the American Society of Clinical Oncology, Chair of the Alliance for Clinical Trials in Oncology. In 2021, she was elected to the National Academy of Medicine, having previously served on the National Academies National Cancer Policy Forum.
-
Challenging the Status Quo in Colorectal Cancer 2024
December 6-8, 2024
-
ESMO 2024 Congress
September 13-17, 2024
-
ASCO Annual Meeting
May 30 - June 4, 2024
-
Yvonne Award 2024
May 31, 2024
-
OncoThon 2024, Online
Feb. 15, 2024
-
Global Summit on War & Cancer 2023, Online
Dec. 14-16, 2023