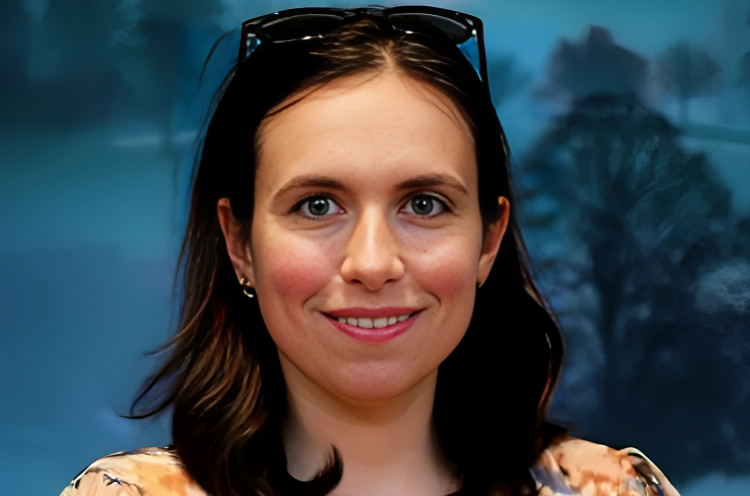
Simona Cristea: Impressive advancement in Computational Pathology
Simona Cristea, Head of Genomics Data Science and AI in the Hale Center for Pancreatic Cancer at Dana-Farber Cancer Institute, shared a post on X:
“Impressive advancement in Computational Pathology.
A new multimodal foundation model by AI4Pathology trained on 47,000 paired histology and genomics, which beautifully shows the multi-modal power of images and DNA and RNA.
Even though patient genomic data is rare, it’s so powerful thread.
First, why is this model so important? To my view, THREADS is the closest we have today to a cancer-level patient-centric foundation model. It beautifully integrates lots of images, DNA and RNA – 3 data modalities providing critical orthogonal information about cancerous tissues.
For some background: Computational Pathology has been really revolutionized by Deep Learning (arguably like no other cancer-related field). It turns out that the usual slides that pathologists read to diagnose and investigate tumors are very “learnable”.
In other words, by ingesting large quantities of cancer slides, Deep Learning models can learn distinguishing features of cancerous conditions. They can then predict, with pretty high accuracy, which (list of) conditions or clinically-relevant features an unseen slide displays.
Examples include:
– cancer type
– point mutations (TP53, BRCA, IDH1 etc)
– treatment status (treated/untreated)
– survival after treatment
– molecularly-defined cancer subtype
– prostate cancer likelihood by scoring
see e.g. TITAN for state-of-the-art.
This space is very active, with a new, bigger (and better) model coming up every month. However, even if the input data for such models is whole tissue slide images (WSI) +/- doctor’s notes – it is known that biology is complex. It’s then likely that some aspects remain unobserved.
This is where multi-modality comes into play (unsurprisingly gaining inspiration from the computer vision space). This is also where genomics comes into play. Genomics data offers a pretty orthogonal perspective to pathology slides when it comes to cancer.
Therefore, integrating genomics with whole-slide imaging data (and also with doctors’ notes) is expected to further boost the performance of such models. However, there’s a catch: genomics data is much more rare and expensive than slides. For this reason, it’s not used too often.
The new algorithm, callee THREADS, is trained on the largest to-date collection of paired histology and genomic and transcriptomic data:
– images: WSI
– transcriptomics: bulk RNAseq
– genomics: DNA (SNVs and CNVs from a targeted panel)
In total, 47,171 paired data points.
The quantity and quality of this data collection are impressive. It was curated from several sources: – Massachusetts General Hospital (14.6%) – Brigham and Women’s Hospital (43.6%) – The Cancer Genome Atlas Program (TCGA, 21.6%) – The Genotype–Tissue Expression (GTEx, 20.2%)
Interestingly, the model encodes the single cell transcriptomic profiles using scGPT by Bo Wang, using the embeddings of the cancer version of the scGPT model, pre-trained on 5.7 million cancer-related cells. The genomics profiles are encoded using an MLP trained from scratch.
The images are encoded in 2 steps:
1. an encoder of a Vision Transformer trained via multimodal learning of millions of image patches and text captions
2. a “slide-level” encoder that uses attention-based modeling to aggregate the patches learned in (1) into a slide-level model
This image shows in more detail the datasets used for model pre-training (a) and, extremely beautifully, the embedding space (TSNE) of the whole-slide images embeddings, clustering really well by primary organ (b).
Each colored dot here is a WSI.
The new algo has been benchmarked on 54 pathology tasks from 23 cohorts using 26,000 WSI from 20 institution. Tasks are split across 4 groups:
– mutation prediction
– clinical subtyping and grading
– immunohistochemistry (IHC) status prediction
– treatment and survival prediction
It performs very well across almost all these tasks, better than previous state of art models.
This comprehensive evaluation of THREADS and other WSI models (PRISM, GIGAPATH, CHIEF) on a large dataset is valuable in an of itself.
purple: THREADS
teal: PRISM
blue: GP
orange: CHIEF
THREADS also performs very well on treatment prediction tasks: predicting treatment response in GBM, ovarian cancer and prostate cancer.
Interestingly, its embeddings can also be used for patient survival prediction, exemplified on pancreatic cancer, colon cancer and head and neck.
THREADS is also evaluated on the same tasks after finetuning. Overall, finetuning leads to an average absolute gain of 2.2% across all 54 tasks (which may not seem much, but it’s a significant gain for the numbers here).
The mutation prediction task benefited most from finetuning.
And now for the coolest part… THREADS can also be prompted with “molecular prompting”.
It finds cases similar to a molecular query, without having seen them before. Here, class-wise molecular prototypes are used for cross-modal slide retrieval and classification.
… and many more interesting details in the THREADS preprint, which is really well written and great to read. I expect this model to be a significant contribution not only to computational pathology, but the way we view cancer as a computational problem.”
-
ESMO 2024 Congress
September 13-17, 2024
-
ASCO Annual Meeting
May 30 - June 4, 2024
-
Yvonne Award 2024
May 31, 2024
-
OncoThon 2024, Online
Feb. 15, 2024
-
Global Summit on War & Cancer 2023, Online
Dec. 14-16, 2023