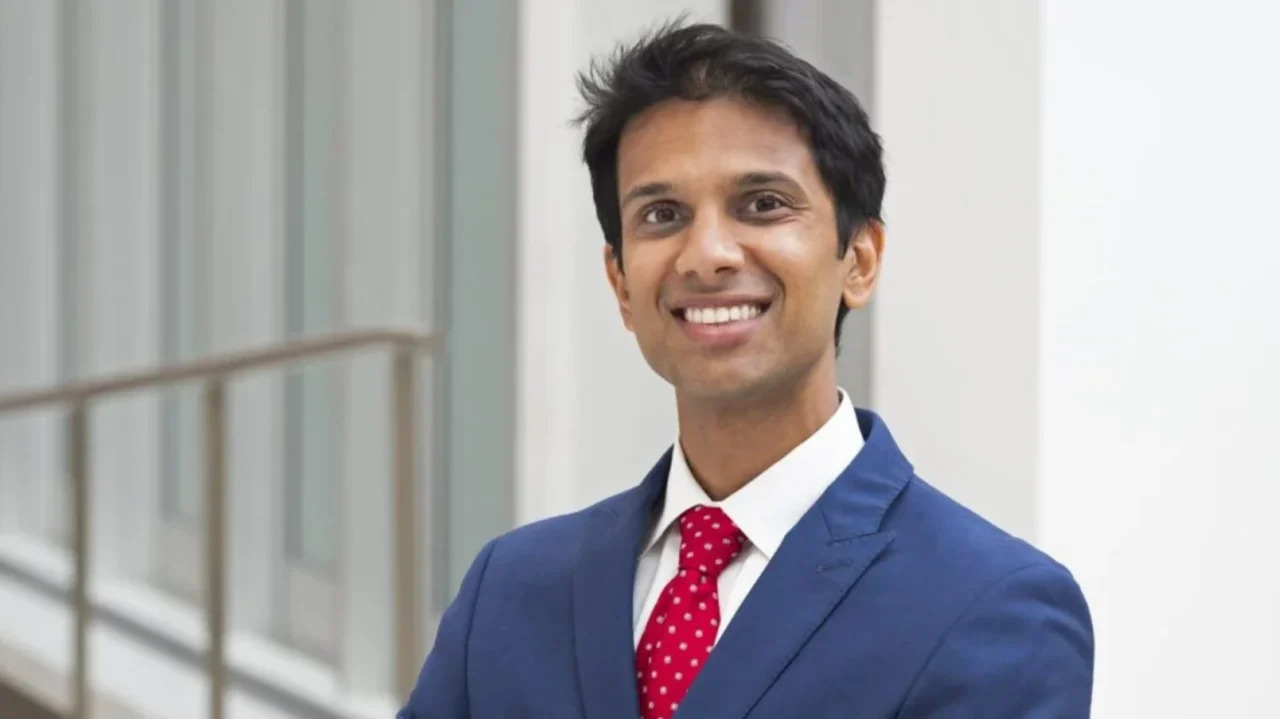
Ravi Parikh: TrialTranslator – a machine learning framework for individualized cancer trial emulation
Ravi B. Parikh, Director of the Human-Algorithm Collaboration Lab (HACLab) at Emory University and Winship Cancer Institute, shared a post on X:
“One of the hardest things I do in oncology clinic is translate results from a clinical trial to the patient in front of me. In Nature Medicine, we introduce TrialTranslator, a machine learning framework for individualized cancer trial emulation.
TLDR: By emulating 11 RCTs in >150K real-world patients, we show that survival results from trials generalize poorly to real-world pts, but in predictable ways. ML framework and web interface trialtranslator.com to facilitate individualized estimation of tx benefit.
Why are trials difficult to generalize? For one, trial eligibility criteria are strict; James Zou and others have shown that relaxing eligibility criteria in trials would likely still preserve treatment effect seen in trials.
But even with “relaxed” eligibility criteria, the type of patient that participates in a trial is different from a typical patient in ways that we can and can’t measure. Maybe that’s why real-world survival is so much worse than survival in trials (figure by Gabrielle Rocque below).
Unfortunately, I’m usually limited to using evidence from trial patients to make decisions for real-world patients who may be sicker or otherwise different from a typical trial participant. That often results in overtreatment.
Granular real-world datasets allow for higher-quality emulations. However, key question still remains: Can we identify subgroups of real-world patients in whom trials generalize poorly, to enable better counselling and alternate trial design?
Enter our platform, TrialTranslator. Using longitudinal data from >150K patients with breast, prostate, lung, and colorectal cancers, we first trained multiple cancer-specific prognostic algorithms to predict mortality risk from the time of metastatic diagnosis.
Next, we carefully emulated 11 landmark cancer trials with positive results, matching on granular trial eligibility criteria, and risk-phenotyped patients within emulated cohorts based on ML risk. Finally, we compared results from emulated trials across phenotypes to RCT results.
What did we find? While we successfully emulated top-line results in >90% of trials, survival advantages were 35% lower in real-world populations compared to those in trials. Put another way: the median OS was, on average, 3.0 months shorter in real-world trials than in the RCTs.
Even more interesting: for patients in low-risk phenotypes (representing ~30% of pts), survival times were aligned with RCT results. But for HIGH-risk phenotypes (~30% think sicker individuals), survival benefits were ~6 mos less than RCTs and often meaningless.
Recognizing that readers may want an easy-to-understand interface, we release trialtranslator.com, a research-only interface to explore outputs from individualized trial emulation. FYI we learned a lot about website development through this project!
We conducted extensive semi-synthetic data simulations and other tests to address robustness of results to unmeasured confounding, violation of positivity assumption, missing data, strictness of emulation, and heterogeneity in dosing. Results were all consistent with main results.
Where do we go from here? As real-world data gets more granular, we will generate more granular phenotypes of response. We hope that this platform can move from trial emulation and binary treatment effect estimation to decision support for multi-class treatment selection.
Winship Cancer Institute of Emory University press release here.
Amazing team: Xavier Orcutt (first author), Kan Chen, Ronac Mamtani, co-senior Qi Long. Xavier started this 4 yrs ago as a resident and has uncanny ability to merge clinical insight with superb data science. Big plans ahead to extend this!”
Beau Bosko Toskich, shared this post, adding:
“Trialtranslator.com is a very smart display of how to rethink our interpretation of the legacy clinical trial.
We still use historical oncology trial designs that will struggle to keep up with advancement. Need to adapt.
Granularity > Generalizability.
Phenotype and experience are how decisions are made.
Huge congrats to Ravi B. Parikh and team.”
Authors: Xavier Orcutt et al.
Ravi B. Parikh, MD, MPP, FACP, is a medical oncologist and Director of the Human-Algorithm Collaboration Lab (HACLab) at Emory University and Winship Cancer Institute.
He serves as a Consultant Editor for the Journal of Clinical Oncology, a columnist for Medscape, and is a Senior Clinical Advisor and Board Member at the Coalition to Transform Advanced Care (C-TAC).
His work focuses on developing, implementing, and evaluating AI-driven digital health interventions and value-based care models through observational studies and pragmatic clinical trials.
-
Challenging the Status Quo in Colorectal Cancer 2024
December 6-8, 2024
-
ESMO 2024 Congress
September 13-17, 2024
-
ASCO Annual Meeting
May 30 - June 4, 2024
-
Yvonne Award 2024
May 31, 2024
-
OncoThon 2024, Online
Feb. 15, 2024
-
Global Summit on War & Cancer 2023, Online
Dec. 14-16, 2023