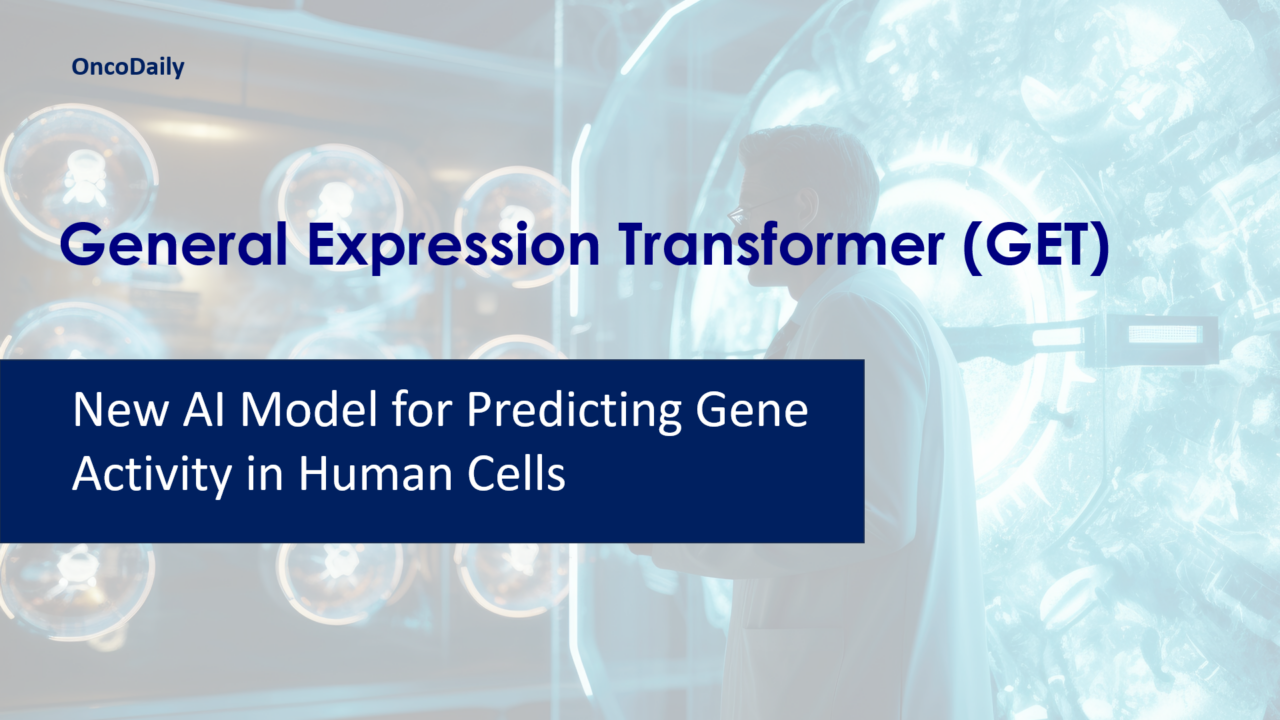
Columbia University researchers have developed new AI Model for Predicting Gene Activity in Human Cells
Researchers at Columbia University Vagelos College of Physicians and Surgeons, led by Dr. Raul Rabadan, have developed a new predictive AI system called the General Expression Transformer (GET) to analyze gene activity in human cells with remarkable accuracy.
This AI model aims to enhance the understanding of cellular mechanisms in both healthy and diseased states. GET is designed to “uncover regulatory grammars” across 213 different human fetal and adult cell types by utilizing chromatin accessibility data alongside genomic sequences. By training on millions of cells collected from normal human tissues, GET has learned how cells typically function, enabling it to predict gene expression patterns effectively in various contexts, including both normal and diseased cells.
“It’s really a new era in biology that is extremely exciting; transforming biology into a predictive science,” – said Raul Rabadan.
On January 8th, 2025, the model and analysis were published in Nature.
A foundation model of transcription across human cell types
Authors: Raul Rabadan et al.
The study introduces the General Expression Transformer (GET), a foundation model designed to unravel the complexities of transcriptional regulation across various human cell types. Transcriptional regulation is important for numerous biological processes, including those related to genetic diseases and cancers.
This regulation is orchestrated by a network of transcription factors (TFs), coactivators, and RNA polymerase II, which interact with regulatory sequences to modulate gene expression. Despite the conserved nature of these interactions, our understanding has often been limited to specific cell types, making it challenging to generalize findings across different contexts.
“Predictive generalizable computational models allow [us] to uncover biological processes in a fast and accurate way. These methods can effectively conduct large-scale computational experiments, boosting and guiding traditional experimental approaches,” – said Rabadan.
GET predicts gene expression in both familiar and novel cell types, demonstrating adaptability to various sequencing platforms and assay types, including tumor cells.
The model not only predicts gene expression but also identifies long-range regulatory elements associated with fetal hemoglobin and their corresponding TFs. The design of GET is based on the principles of transcription regulation, focusing on how genomic regions interact with TFs and how accessible these regions are in specific cell types. This interaction shapes the chromatin environment, which influences how RNA polymerase II drives gene expression.
GET employs a two-stage training process: first, it undergoes self-supervised pretraining to learn the interactions between regulatory elements, followed by fine-tuning to predict gene expression without needing paired expression measurements. In terms of performance, GET has shown impressive accuracy in predicting gene expression for unseen cell type. This performance surpasses that of previous models and emphasizes the importance of DNA sequence specificity in transcription regulation. GET also demonstrates generalizability to adult cell types when trained solely on fetal data.
GET’s ability for zero-shot prediction was validated using a lentivirus-based massively parallel reporter assay (lentiMPRA), where it effectively identified regulatory elements in a cell-type-specific context without prior exposure to relevant data. This capability allows GET to predict regulatory activity across different genetic sequences.The model interpretation techniques used in GET enable researchers to derive scores indicating the contribution of specific regions or motifs to gene expression across various cell types.
“The vast majority of mutations found in cancer patients are in so-called dark regions of the genome. These mutations do not affect the function of a protein and have remained mostly unexplored. The idea is that using these models, we can look at mutations and illuminate that part of the genome,” – said Rabadan.
GET has proven effective in discovering coregulating TFs by analyzing correlations between motifs. The model’s causal discovery algorithms have uncovered both known and novel interactions between TFs, confirming its potential for elucidating complex regulatory mechanisms. GET represents an advancement in transcriptional modeling, offering insights into regulatory elements, upstream regulators, and TF interactions across diverse human cell types. Its broad applicability positions it as a valuable tool for understanding gene regulation in health and disease contexts. Future enhancements could further integrate various biological data layers to provide an even more comprehensive view of transcriptional regulation dynamics.
Further Reading:
Tempus AI Introduced xH: Its First Whole-Genome Sequencing Test
-
ESMO 2024 Congress
September 13-17, 2024
-
ASCO Annual Meeting
May 30 - June 4, 2024
-
Yvonne Award 2024
May 31, 2024
-
OncoThon 2024, Online
Feb. 15, 2024
-
Global Summit on War & Cancer 2023, Online
Dec. 14-16, 2023