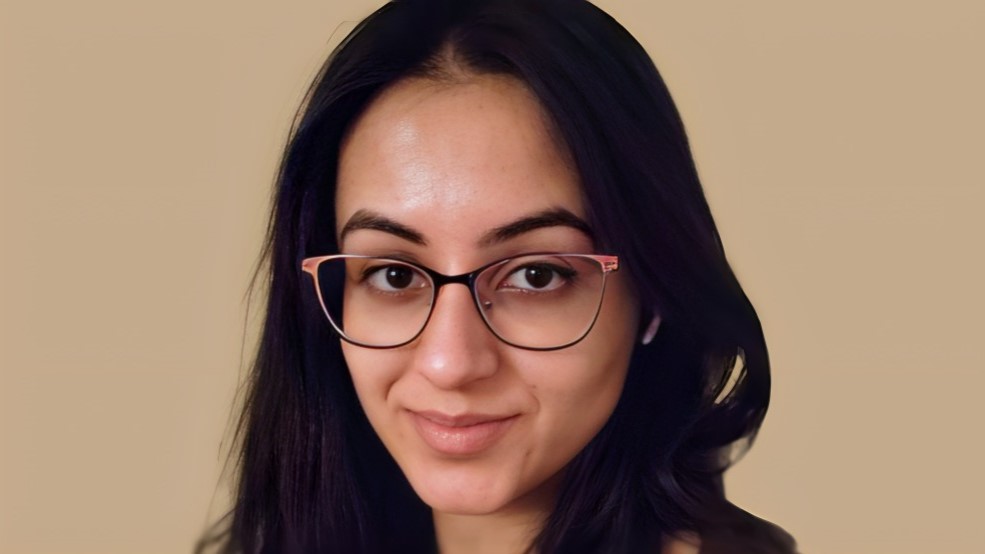
Sanna Madan: Can we engineer smarter CAR-T cells that target cancer with logical precision?
Sanna Madan, PhD Student at the National Cancer Institute (NCI), shared a paper she and her colleagues authored on X:
“Can we engineer smarter CAR-T cells that target cancer with logical precision?
Thrilled to share the heart of my PhD: LogiCAR designer, a framework that identifies logic-gated antigen circuits for next-generation cell therapies. With Tiangen Chang.
CAR therapies have yielded tremendous clinical success, especially against malignancies of B-cell origin. However, their success remains limited in solid tumors when using single-antigen targets due to tumor antigen heterogeneity and off-tumor toxicities.
To overcome these challenges, researchers are developing next-gen CAR designs targeting multiple antigens with Boolean logic gates (AND, OR, NOT). These circuits improve efficacy by overcoming heterogeneity, and safety via increased specificity [Williams et al., Science ’21]
Given this, we asked: can we systematically harness patient tumor single-cell data to identify logic-gated antigen combinations (i.e., “circuits”) for designing CAR therapies that precisely target cancer cells while sparing healthy tissues?
To address this challenge, we developed LogiCAR designer: a computational framework that identifies logic-gated antigen combinations from single-cell data. It optimizes for circuits that target the majority of cancer cells while sparing healthy tissues.
LogiCAR designer uses a genetic algorithm to discover near-optimal antigen circuits with unprecedented scale and efficiency. It scales to combinations of up to five genes – a feat not previously accomplished to our knowledge.
LogiCAR designer is highly efficient. Runtime scales linearly with gene combination size vs. exponentially with exhaustive search. Convergence is independent of input size. For 3-gene circuits, LogiCAR runs in <1 hour on a typical laptop vs. >450 days for exhaustive search.
To test LogiCAR designer on a large scale, we assembled a first-of-its-kind breast cancer dataset comprising ~2 million cells (>620k tumor cells) from 342 patient samples, consisting of 15 public cohorts and 2 in-house cohorts.
For safety evaluation, we used ~700k cells across 31 healthy tissues from the Human Protein Atlas. In our optimization process, we require LogiCAR-identified circuits to meet a stringent safety threshold (set to >90% of healthy cells spared).
We first optimized LogiCAR designer on the 15 public discovery cohorts to identify “shared circuits” across patients.
The results were striking: LogiCAR-identified circuits outperform clinical CAR targets and previously identified circuits from two state-of-the-art studies.
Remarkably, LogiCAR-identified circuits maintained their superior performance in two independent validation cohorts:
1) a multi-ethnic 82-patient cohort spanning all breast cancer subtypes that we generated here, and
2) a 35-patient TNBC cohort.
How might LogiCAR circuits translate to outcomes?
We mapped efficacy to treatment responses. In oncology, tumor radius reductions (10%, 30%) yield volume reductions (27%, 66%) – defining minimal and partial responses. We define >99% volume reduction as complete response.
The results are promising: e.g., our top general circuit ‘GABRP OR PRLR OR VTCN1’ could achieve minimal response in 85% of patients, partial response in 50% of patients, and complete response in 16% of patients – far outperforming existing approaches.
But, can we improve upon the estimated 16% complete response rate?
We try another strategy: matching each patient to the best possible general LogiCAR circuit. This strategy can boost the predicted complete response rate to 23%.
However, even optimized shared circuits can’t achieve optimal targeting for all patients. Focusing on our diverse cohort 1, we studied LogiCAR designer’s ability to identify individualized CAR circuits *optimized to each patient’s tumor.*
Strikingly, personalized LogiCAR circuits provide estimated tumor-targeting efficacy tantamount to complete clinical response in 76% of patients and at least partial response for all patients! If achieved clinically, these response rates would revolutionize cancer treatment.
Personalized LogiCAR circuits could deliver precision-engineered CAR therapies with unprecedented efficacy by addressing each patient’s unique tumor heterogeneity.
In sum, LogiCAR designer offers a data-driven framework to facilitate the rational design of safer and more effective CAR immunotherapies for cancer, addressing the fundamental challenges of both inter- and intra-tumor heterogeneity.
While we focused on breast cancer, LogiCAR designer can be readily applied to any cancer type and even beyond – such as to autoimmune conditions or aging-related pathologies, where targeted cell therapies show increasing promise.
Our ultimate vision is to realize rationally designed, intelligent cell therapies.
It’s a pleasure to co-lead this work with the amazing Tiangen Chang. Huge thank you to my mentors Eytan Ruppin and Alejandro Schäffer for their invaluable guidance.
And to our amazing co-authors who made this possible: Alexandra Harris, Huaitian Liu, Andrew Martinez, Saugato Rahman Dhruba, Binbin Wang, Sheila Rajagopal, Sanju Sinha, Aravind Srinivasan, Simon Knott, Shahin Sayed, Francis Makokha, Chi-Ping Day, Gretchen Gierach, Stefan Ambs.
Check out our bioRxiv manuscript for even more insights.
Authors: Sanna Madan et al.
-
Challenging the Status Quo in Colorectal Cancer 2024
December 6-8, 2024
-
ESMO 2024 Congress
September 13-17, 2024
-
ASCO Annual Meeting
May 30 - June 4, 2024
-
Yvonne Award 2024
May 31, 2024
-
OncoThon 2024, Online
Feb. 15, 2024
-
Global Summit on War & Cancer 2023, Online
Dec. 14-16, 2023