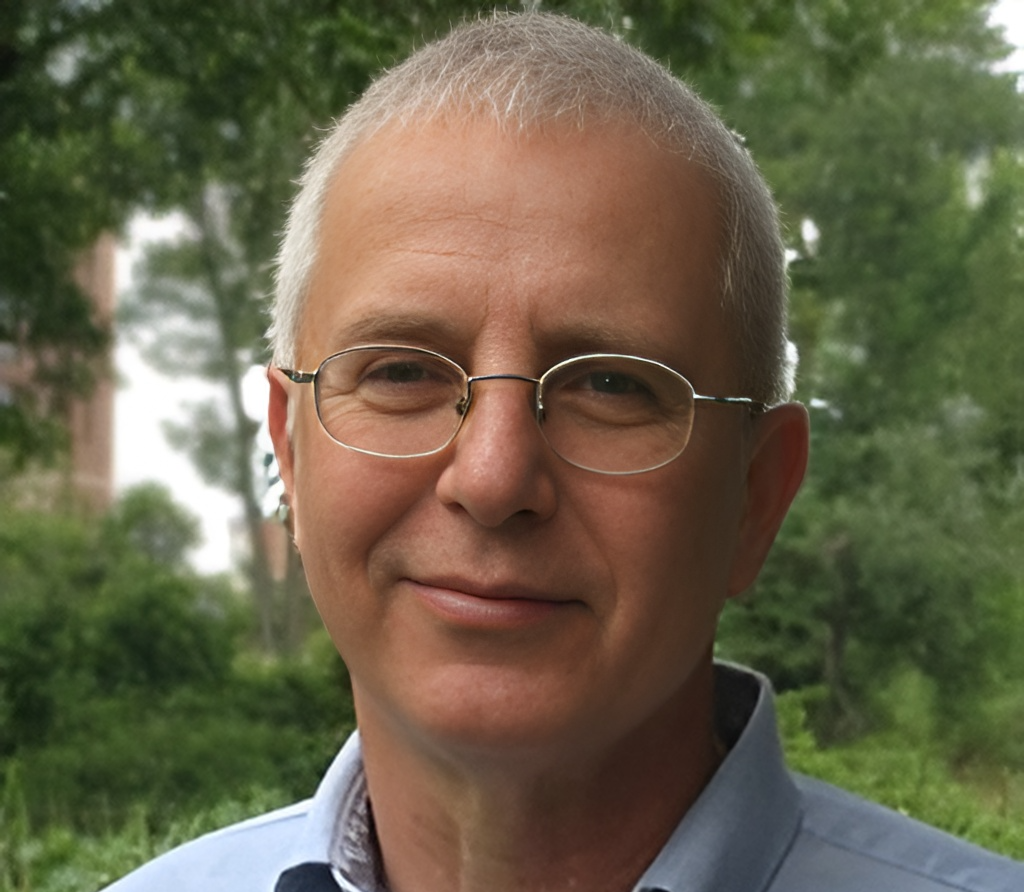
Eytan Ruppin: Analyzing Tumor Microenvironment using bulk transcriptomics with Machine Learning
Eytan Ruppin, Chief of the Cancer Data Science Lab of the National Institute of Health, shared a post on X:
“Excited to share our latest work on analyzing Tumor Microenvironment using bulk transcriptomics with Machine Learning, now out on bioRxiv..
We present DECODEM, a generic ML framework to systematically assess how different cells in the Tumor Microenvironment contribute to patient response to a given treatment. DECODEM leverages cellular deconvolution to circumvent the need for single-cell data.
We further present DECODEMi (i = interaction), an extension of DECODEM to incorporate inferred immune cell-cell interactions for clinical response prediction, thereby identifying the most predictive interactions in Tumor Microenvironment.
Applying DECODEM on three cohorts of Breast Cancer patients undergoing Chemotherapy reveals that gene expression of specific immune (myeloid, plasmablasts, B-cells) and stromal (endothelial, normal epithelial, CAFs) cells are highly predictive of patient response.
We demonstrate the complementary impacts of these cell types in chemotherapy response via building multi-cell-ensemble predictors, remarkably finding a immune–stromal three-cell-ensemble of endothelial, myeloid, and plasmablasts achieving highest accuracy across cohorts.
DECODEM captures treatment-invariant properties of tumor microenvironment, illustrated by its ability to stratify: (a) patient response to chemotherapy and immunotherapy from single cell transcriptomics and (b) patient survival in TCGA.
Employing DECODEMi with a set of Breast Cancer-specific ligand-receptor interactions, we identify key immune cell-cell interactions from bulk transcriptomics that are strongly associated with chemotherapy response, validated on both bulk and single-cell.
In sum, DECODEM and DECODEMi are two data-driven tools facilitating systematic exploration of Tumor Microenvironment from widely available bulk transcriptomics to quantify the association with different cancer treatment outcomes, possibly across many cancer indications.”
Read further.
Source: Eytan Ruppin/X
-
Challenging the Status Quo in Colorectal Cancer 2024
December 6-8, 2024
-
ESMO 2024 Congress
September 13-17, 2024
-
ASCO Annual Meeting
May 30 - June 4, 2024
-
Yvonne Award 2024
May 31, 2024
-
OncoThon 2024, Online
Feb. 15, 2024
-
Global Summit on War & Cancer 2023, Online
Dec. 14-16, 2023