Douglas Flora, Executive Medical Director of Oncology Services at St. Elizabeth Healthcare, shared a post on LinkedIn:
“Cracking Cancer’s Code: The Unlikely Mathematicians Who Started It All
Before AI became the buzzword reshaping Medicine, it was an idea forged in the urgency of war. The story of AI didn’t start in Silicon Valley or a cutting edge research lab – it begins with a group of mathematicians at Bletchley Park, racing against time to break the Nazi Enigma code. Their breakthroughs in computing laid the foundation for something much bigger: computers that could think, learn, and ultimately help us decode cancer.
In this draft of Chapter 3 of my upcoming book, Ctrl+Alt+Cure: Rebooting Cancer Care, I trace this unexpected path from wartime cryptography to modern-day oncology, showing how the first sparks of AI were lit in one of history’s most unlikely places.
I hope you enjoy it – these are fun to research and write! Let me know what you think. In Chapter 4, I’ll introduce you to the technologies driving these advances and give a primer on AI terms you need to know and why.
Code Breakers 2.0: From Hitler’s Cipher to Cancer’s Source Code
“The real voyage of discovery consists not in seeking new landscapes, but in having new eyes.”– Marcel Proust
In the gathering dusk of a winter evening in 1939, a young mathematician walked the grounds of an elegant Victorian estate in Buckinghamshire, England. The gravel crunched beneath his feet as his mind raced through possibilities – not of war strategy or military tactics, but of patterns, algorithms, and the fundamental nature of computation itself. Alan Turing, already known in academic circles for his groundbreaking work on computational theory,(1) had arrived at Bletchley Park with an audacious idea: machines could think.
The manor house before him, its red brick facade still elegant despite its hasty conversion into Britain’s top-secret code-breaking center, would become the unlikely birthplace of artificial intelligence. Here, amid the urgent clatter of prototype computers and the whispered conversations of mathematicians, the first seeds of machine learning were planted—seeds that would, three-quarters of a century later, bloom into technologies with the potential to revolutionize how we understand and treat cancer.
The urgency was palpable. Each day, German U-boats were sinking Allied ships in the Atlantic, guided by encrypted messages that the British could intercept but not decode. With its intricate rotors and labyrinth of electrical circuits, the Enigma machine could scramble text in approximately 159 quintillion different ways (2)—a number so vast it defied human comprehension. The Germans believed, with mathematical certainty backed by their finest cryptographers, that breaking Enigma would take more than a human lifetime.(3)
But Turing saw something different. Where others saw an insurmountable wall of complexity, he envisioned a machine that could think systematically through possibilities, learning from each attempt and eliminating impossible combinations with ruthless logical efficiency.
His solution – the Bombe machine – would become one of history’s first examples of mechanical intelligence augmenting human cognition.(4)
Standing over six feet tall and weighing more than a ton, the Bombe worked backward through Enigma’s encryption, simultaneously testing thousands of possible settings. It was, in essence, the world’s first practical implementation of parallel processing, a concept that would become crucial to modern artificial intelligence. (5)
As oncologists today grapple with the staggering complexity of cancer – its countless genetic mutations, its ability to evolve and adapt, its unique presentation in each patient – we find ourselves facing a challenge not unlike Turing’s. The amount of data a single cancer patient generates, from genomic sequences to metabolic profiles, from cellular imaging to treatment responses, exceeds what any human mind can fully comprehend.(6)
Just as Turing’s Bombe machine helped crack patterns that were humanly impossible to process, modern artificial intelligence promises to decode cancer’s cryptic messages written in the language of genes, proteins, and cellular signals.
The parallel is more than metaphorical. The mathematical principles Turing developed at Bletchley Park – principles of pattern recognition, learning from data, and systematically eliminating impossible solutions – lie at the heart of today’s most sophisticated cancer-detecting AI systems(7). When a deep learning algorithm scans a mammogram for subtle signs of breast cancer, achieving accuracy rates that match or exceed human radiologists,(8) or when a neural network analyzes pathology slides with superhuman precision,(9) it is building upon foundations laid in that Victorian manor house during humanity’s darkest hours.
The Birth of AI: The Dartmouth Summer Conference
On a warm summer day in 1956, amid the verdant grounds of Dartmouth College, an eclectic group of scientists gathered to explore an idea so audacious it seemed to border on science fiction. Their proposal, outlined in a modest three-page document, began with a statement of breathtaking ambition:
“Every aspect of learning or any other feature of intelligence can, in principle, be so precisely described that a machine can be made to simulate it.”(10)
The Dartmouth Summer Research Project on Artificial Intelligence marked the moment when scattered threads of computational theory, cognitive science, and mathematical logic were first woven into a coherent vision of machine intelligence.
The attendees included John McCarthy, whose precise mathematical mind would help define the field’s theoretical foundations; Marvin Minsky, whose theories of neural computation would eventually influence modern deep learning; Claude Shannon, whose information theory provided the mathematical framework for understanding both genetic codes and computer algorithms; and Herbert Simon, whose work on decision-making would revolutionize our understanding of human problem-solving.(11)
In the intimate setting of the Dartmouth campus, these pioneers spent their days in intense discussion, their blackboards filled with equations and diagrams that would lay the groundwork for artificial intelligence as a formal field of study. They debated the nature of human intelligence, the possibilities of machine learning, and the fundamental relationships between information, computation, and understanding.(12)
The first practical medical applications of AI emerged from this period of early optimism. DENDRAL, developed at Stanford in 1965, helped chemists identify unknown organic molecules by applying rule-based reasoning to mass spectrometry data.(13) Though primitive by today’s standards, it demonstrated how machines could augment human expertise in analyzing complex biological data – a direct ancestor of today’s AI systems that analyze molecular pathways in cancer cells.
Even more influential was MYCIN, developed in the early 1970s at Stanford. It was one of the first expert systems designed specifically for medical diagnosis, focusing on blood infections and selecting appropriate antibiotics.(14) In a rigorous evaluation, MYCIN’s treatment recommendations achieved accuracy rates that matched or exceeded those of practicing physicians, correctly identifying pathogens and suggesting appropriate treatments in cases that often puzzled human experts.(15)
These early systems’ challenges would presage many difficulties in implementing modern medical AI. MYCIN, despite its impressive performance, was never implemented in clinical practice. The reasons – questions of liability, the difficulty of integrating systems into clinical workflows, and doctors’ understandable reluctance to trust computer-generated recommendations – remain relevant to today’s efforts to implement AI in oncology. (16)
Yet the most significant development of this era wasn’t technological but conceptual. The Dartmouth conference established artificial intelligence as a field defined not by specific technologies but by an ambitious vision: that human intelligence, in all its complexity, could be understood as a form of information processing. This insight would prove transformative for both computer science and medicine.
The early AI researchers’ fundamental insight – that intelligence could be reduced to computable processes – has found its ultimate expression in modern oncology. When deep learning systems analyze pathology slides with superhuman accuracy (17) or when transformer models predict protein structures crucial for drug development,(18) they validate that original vision, even if the specific technologies would be unrecognizable to those pioneers.
AI vs. the Human Mind: Moments of Truth
On May 11, 1997, in a darkened room on the 35th floor of the Equitable Center in Manhattan, Garry Kasparov stared at a chessboard in disbelief. The world chess champion had just lost to IBM’s Deep Blue, marking the first time a computer had defeated a reigning world champion under standard chess tournament conditions.(19) The machine’s victory sent shockwaves through the scientific community and beyond, challenging long-held assumptions about the uniqueness of human intelligence.
Deep Blue’s triumph came not through intuition or creativity – qualities long considered uniquely human – but through raw computational power. The system could evaluate 200 million chess positions per second, approaching complex decisions through sheer calculational force.(20) Yet this victory, while historic, represented what AI researchers call “narrow intelligence” – supremacy in a domain with clearly defined rules and boundaries. Chess, despite its complexity, is ultimately a finite game with perfect information.
The parallel to early attempts at AI-driven cancer diagnosis is striking. First-generation diagnostic systems, like Deep Blue, relied on brute-force application of predefined rules. They could process vast amounts of data but lacked the flexibility to handle ambiguous or incomplete information – a crucial limitation in medical applications, where uncertainty is the norm rather than the exception.(21)
The next landmark would come fourteen years later, when IBM’s Watson competed on the television quiz show Jeopardy! Facing champions Ken Jennings and Brad Rutter in February 2011, Watson demonstrated something far more sophisticated than Deep Blue’s brute-force calculation: understanding natural language, processing ambiguous information, and generating appropriate real-time responses.(22)
Watson’s victory was impressive not just for its breadth of knowledge but also for its ability to parse the show’s complex wordplay and contextual clues. The system combined natural language processing, information retrieval, and machine learning in ways that seemed to approach human-level understanding.(23) IBM quickly recognized the potential medical applications of this technology, particularly in oncology, where clinicians must constantly synthesize new research, clinical data, and treatment guidelines.
By 2012, IBM had partnered with Memorial Sloan Kettering Cancer Center to adapt Watson’s capabilities to oncology.
The vision was compelling: Watson could read and understand millions of pages of medical literature, clinical trials, and patient records, synthesizing this vast knowledge to support clinical decision-making.(24) Early results showed promise, with Watson demonstrating the ability to suggest treatment options that matched those of human tumor boards with high consistency.(25)
However, the reality of clinical medicine proved more complex than a quiz show format. Watson struggled with the messiness of real-world medical data, the ambiguities of clinical practice, and the need to integrate multiple types of information – imaging, genomics, clinical history – in ways that often defied straightforward algorithmic analysis.(26)
The lesson was clear: success in a structured environment didn’t necessarily translate to the nuanced world of clinical medicine.
The next breakthrough would come from an unexpected direction: the ancient game of Go. While chess has approximately 10^120 possible game positions, Go has 10^170 – more than the number of atoms in the universe.(27) Where Deep Blue could succeed through brute-force calculation, any system hoping to master Go would need something closer to genuine intelligence.
DeepMind’s AlphaGo, unveiled in 2016, represented a quantum leap in artificial intelligence. The system combined neural networks with reinforcement learning – essentially, learning from experience. In a historic match played against Lee Sedol, one of the world’s strongest Go players, AlphaGo demonstrated superhuman performance and genuinely creative play.(28)
The system’s most famous moment came in Game 2, with Move 37 – a play so unexpected and sophisticated that it initially stunned both human experts and the watching world. Go professionals, who had dismissed AlphaGo’s chances before the match, found themselves studying its games for new insights into their ancient art.(29) The machine wasn’t just winning; it was teaching.
AlphaGo’s approach to Go mirrored emerging trends in medical AI. Rather than relying on predetermined rules or brute-force calculation, modern medical AI systems use deep learning and reinforcement learning to discover patterns in complex data. They can identify subtle indicators of disease in medical images, predict patient outcomes from complex clinical data, and suggest novel treatment approaches.(30)
The evolution from Deep Blue to AlphaGo parallels the evolution of medical AI: from rigid, rule-based systems to flexible learning algorithms that can discover new patterns and possibilities. But perhaps the most important lesson from these landmark matches isn’t about machine capability – it’s about human-machine collaboration.
In the years following his loss to Deep Blue, Kasparov pioneered the concept of “centaur chess,” where humans and computers play as partners rather than opponents. These human-AI teams consistently outperform both solo humans and solo computers.(31) Similarly, studies have demonstrated that oncologists working with AI support achieve higher diagnostic accuracy than either humans or machines alone(32), suggesting that the future of cancer care lies not in artificial intelligence replacing human doctors but in a sophisticated partnership between human intuition and machine analysis.
The Cambrian Explosion: From Machine Learning to Transformers
In evolutionary history, there is a moment known as the Cambrian Explosion – a period when life suddenly and dramatically diversified into countless new forms. In the history of artificial intelligence, the years between 2012 and 2024 mark a similar explosion of possibility. This era witnessed not just incremental improvements but a fundamental transformation in how machines learn and reason.
The catalyst came in 2012 at a computer vision competition in Silicon Valley. A neural network called AlexNet demonstrated unprecedented accuracy in image recognition, cutting the error rate nearly in half compared to traditional approaches.(33) The system’s success rested on two key developments: deep learning architectures that could automatically discover complex patterns in data and graphics processing units (GPUs) that provided the raw computational power to train them.
For oncology, this breakthrough had immediate implications. Cancer diagnosis often begins with image analysis – mammograms, pathology slides, CT scans – and deep learning proved remarkably adept at this task. By 2017, these systems matched or exceeded human performance in detecting various types of cancer, from melanoma to breast cancer.(34)
But the real revolution wasn’t just in performance but in how these systems learned.
Traditional computer vision relied on human-engineered features – specific patterns that programmers told the computer to look for. In contrast, deep learning discovered its features through exposure to millions of examples. When researchers examined what these networks had learned, they found something remarkable: they had independently discovered many of the same visual patterns that human pathologists use, plus others too subtle for human perception.(35)
Yet image analysis was just the beginning. The real paradigm shift came in 2017 with the publication of “Attention Is All You Need” by Vaswani and colleagues.(36) This paper introduced the Transformer architecture, a new way of processing sequential data that would revolutionize not just natural language processing but also our understanding of biological sequences.
The key insight of transformers was the concept of “attention” – the ability to weigh the importance of different parts of the input when making predictions. Just as a human pathologist knows which parts of a tissue sample are most relevant for diagnosis, transformer models could learn which elements of complex biological data are most significant for understanding disease.
This capability proved transformative when applied to molecular biology.
In 2020, DeepMind’s AlphaFold2, based on transformer architecture, achieved what many had considered impossible: accurate prediction of protein structures from amino acid sequences.(37)
This breakthrough offered new ways to understand cancer at its molecular level, providing insights into how mutations affect protein function and suggesting novel therapeutic targets. This concept will be discussed in much greater detail in several chapters later.
The Future: AI in Oncology and Beyond
In the quiet halls of modern cancer centers, artificial intelligence now works silently alongside human caregivers. Algorithms analyze streaming data from patient monitors, scan pathology slides with superhuman precision, and process genetic sequences to identify therapeutic targets.
This is not the AI of science fiction – not the sentient computers that Alan Turing imagined might one day pass his famous test. Instead, it is something perhaps more profound: a new kind of intelligence that augments rather than replaces human capability, that sees patterns we cannot see, and processes complexities we cannot hold in our minds.
The transformation of cancer care through AI has been both more subtle and more fundamental than early pioneers might have imagined.
Modern diagnostic processes seamlessly integrate human and artificial intelligence. AI systems analyze medical histories, looking for subtle patterns that might escape human notice. Advanced imaging systems, guided by neural networks, provide unprecedented detail about potential tumors. Genetic sequencing, interpreted by transformer models, reveals the molecular signatures of disease.(38)
Perhaps most importantly, AI has transformed drug discovery. Traditional development of cancer therapeutics was a painfully slow process of trial and error. AI has revolutionized this landscape through what researchers call “in silico discovery” – the ability to simulate molecular interactions and predict drug effectiveness before a single physical experiment is conducted.(39)
Yet challenges remain. Integrating AI into cancer care has raised new ethical questions and exposed new vulnerabilities. Issues of data privacy, algorithmic bias, and the digital divide between wealthy and poor healthcare systems have become urgent concerns.(40) The challenge of explaining AI decisions – what computer scientists call the “black box problem” – has taken on new urgency in medical applications.(41)
Looking ahead, the frontier of AI in oncology continues to expand. Researchers are developing “digital twins” – detailed computational models of individual patients that can simulate treatment responses and predict complications before they occur.(42) New approaches to quantum computing promise to unlock even greater capabilities in molecular modeling and drug design.(43)
As we look back to Turing’s walks through the grounds of Bletchley Park, we can see how far we’ve come – and how far we still have to go. The machines he imagined have become a reality, though not in the way he envisioned. They don’t think like humans; they think differently, and in doing so, they expand the boundaries of human capability.
Yet perhaps the most profound change has been in how we think about cancer itself. Through the lens of artificial intelligence, we have come to see cancer not as a single disease to be “cured” but as a complex system of cellular processes to be understood and managed. This shift in perspective – from warfare to systems management – may prove to be AI’s most lasting contribution to oncology.
Ultimately, the story of AI in oncology is not about machines replacing humans but about a new kind of collaboration between humans and artificial intelligence. As Topol argues in his seminal work on medical AI, it is about combining the pattern-recognition capabilities of neural networks with the contextual understanding of human clinicians, the raw computational power of modern computers with the wisdom accumulated through centuries of medical practice.(44)
The future of cancer care lies not in artificial intelligence alone but in the synthesis of human and machine intelligence – each complementing the other’s strengths and compensating for the other’s weaknesses. In this synthesis lies our best hope for understanding and treating cancer, not as a single enemy to be vanquished but as a complex challenge to be met with all the intelligence – both natural and artificial – that we can bring to bear.
The story of AI’s evolution from Turing’s earliest insights to today’s sophisticated neural networks reads like a scientific thriller – full of breakthrough moments, unexpected twists, and profound implications for human health. But to understand how these tools will ultimately help us cure cancer, we must first master their language.
Just as early anatomists needed to map the human body before modern surgery became possible, we need to build a new kind of literacy – one that bridges the worlds of computation and medicine.
In the next chapter, we’ll decode the essential vocabulary and concepts that power these revolutionary tools. Consider this your initiation into a new way of thinking about cancer and computation, where algorithms become as fundamental as antibodies and data flow as vital as blood.
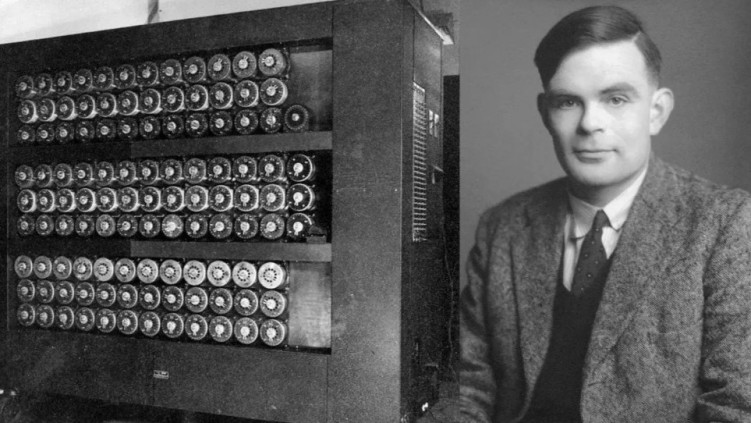
REFERENCES
1. Copeland BJ. Alan Turing: Pioneer of the Information Age. Oxford University Press; 2012.
2. Smith M. The Secrets of Bletchley Park’s Codebreaking Computers. Oxford University Press; 2014.
3. Hinsley FH, Stripp A. Codebreakers: The Inside Story of Bletchley Park. Oxford University Press; 2001.
4. Copeland BJ, et al. Colossus: The Secrets of Bletchley Park’s Codebreaking Computers. Oxford University Press; 2006.
5. Campbell M, Hoane Jr AJ, Hsu F. Deep Blue. Artif Intell. 2002;134(1-2):57-83.
6. Topol EJ. High-performance medicine: the convergence of human and artificial intelligence. Nat Med. 2019;25(1):44-56.
7. Hinton G. Deep learning—a technology with the potential to transform health care. JAMA. 2018;320(11):1101-1102.
8. McKinney SM, Sieniek M, Godbole V, et al. International evaluation of an AI system for breast cancer screening. Nature. 2020;577(7788):89-94.
9. Esteva A, Kuprel B, Novoa RA, et al. Dermatologist-level classification of skin cancer with deep neural networks. Nature. 2017;542(7639):115-118.
10. McCarthy J, Minsky ML, Rochester N, Shannon CE. A proposal for the Dartmouth summer research project on artificial intelligence. AI Magazine. 2006;27(4):12-14.
11. McCorduck P. Machines Who Think: A Personal Inquiry into the History and Prospects of Artificial Intelligence. A.K. Peters; 2004.
12. Nilsson NJ. The Quest for Artificial Intelligence: A History of Ideas and Achievements. Cambridge University Press; 2009.
13. Lindsay RK, Buchanan BG, Feigenbaum EA, Lederberg J. DENDRAL: a case study of the first expert system for scientific hypothesis formation. Artif Intell. 1993;61(2):209-261.
14. Shortliffe EH. Computer-Based Medical Consultations: MYCIN. Elsevier; 1976.
15. Yu VL, Buchanan BG, Shortliffe EH, et al. Evaluating the performance of a computer-based consultant. Computer Programs in Biomedicine. 1979;9(1):95-102.
16. Miller RA. Medical diagnostic decision support systems–past, present, and future. J Am Med Inform Assoc. 1994;1(1):8-27.
17. Cruz-Roa A, Gilmore H, Basavanhally A, et al. Accurate and reproducible invasive breast cancer detection in whole-slide images. NPJ Breast Cancer. 2017;3:31.
18. Jumper J, Evans R, Pritzel A, et al. Highly accurate protein structure prediction with AlphaFold. Nature. 2021;596(7873):583-589.
19. Campbell M, Hoane Jr AJ, Hsu F. Deep Blue. Artif Intell. 2002;134(1-2):57-83.
20. IBM Research. Deep Blue: An Artificial Intelligence Milestone. IBM J Res Dev. 2001;45(2):206-209.
21. Shortliffe EH, Sepúlveda MJ. Clinical decision support in the era of artificial intelligence. JAMA. 2018;320(21):2199-2200.
22. Ferrucci D, Brown E, Chu-Carroll J, et al. Building Watson: An overview of the DeepQA project. AI Magazine. 2010;31(3):59-79.
23. High R. The era of cognitive systems: An inside look at IBM Watson and how it works. IBM Redbooks. 2012.
24. Somashekhar SP, Sepúlveda MJ, Puglielli S, et al. Watson for Oncology and breast cancer treatment recommendations: agreement with an expert multidisciplinary tumor board. Ann Oncol. 2018;29(2):418-423.
25. Nishikawa RM, Huynh PT, Xue J, et al. Artificial intelligence in clinical decision support: challenges and opportunities. J Am Coll Radiol. 2019;16(9):1323-1325.
26. Strickland E. IBM Watson, heal thyself: How IBM overpromised and underdelivered on AI health care. IEEE Spectrum. 2019;56(4):24-31.
27. Silver D, Schrittwieser J, Simonyan K, et al. Mastering the game of Go without human knowledge. Nature. 2017;550(7676):354-359.
28. Silver D, Huang A, Maddison CJ, et al. Mastering the game of Go with deep neural networks and tree search. Nature. 2016;529(7587):484-489.
29. Wang FY, Zhang JJ, Zheng X, et al. Where does AlphaGo go: from church-Turing thesis to AlphaGo thesis and beyond. IEEE/CAA Journal of Automatica Sinica. 2016;3(2):113-120.
30. Yu KH, Beam AL, Kohane IS. Artificial intelligence in healthcare. Nature Biomedical Engineering. 2018;2(10):719-731.
31. Kasparov G. Deep Thinking: Where Machine Intelligence Ends and Human Creativity Begins. PublicAffairs; 2017.
32. McKinney SM, Sieniek M, Godbole V, et al. International evaluation of an AI system for breast cancer screening. Nature. 2020;577(7788):89-94.
33. Krizhevsky A, Sutskever I, Hinton GE. ImageNet classification with deep convolutional neural networks. Commun ACM. 2017;60(6):84-90.
34. Esteva A, Kuprel B, Novoa RA, et al. Dermatologist-level classification of skin cancer with deep neural networks. Nature. 2017;542(7639):115-118.
35. Cruz-Roa A, Gilmore H, Basavanhally A, et al. Accurate and reproducible invasive breast cancer detection in whole-slide images. NPJ Breast Cancer. 2017;3:31.
36. Vaswani A, Shazeer N, Parmar N, et al. Attention is all you need. Advances in Neural Information Processing Systems. 2017;30:5998-6008.
37. Jumper J, Evans R, Pritzel A, et al. Highly accurate protein structure prediction with AlphaFold. Nature. 2021;596(7873):583-589.
38. Zou J, Huss M, Abid A, et al. A primer on deep learning in genomics. Nature Genetics. 2019;51(1):12-18.
39. Fleming N. How artificial intelligence is changing drug discovery. Nature. 2018;557(7706): S55-S57.
40. Char DS, Shah NH, Magnus D. Implementing machine learning in health care—addressing ethical challenges. N Engl J Med. 2018;378(11):981-983.
41. Rudin C. Stop explaining black box machine learning models for high stakes decisions and use interpretable models instead. Nat Mach Intell. 2019;1(5):206-215.
42. Ramesh AN, Kambhampati C, Monson JRT, Drew PJ. Artificial intelligence in medicine. Ann R Coll Surg Engl. 2004;86(5):334-338.
43. Cao Y, Romero J, Olson JP, et al. Quantum chemistry in the age of quantum computing. Chem Rev. 2019;119(19):10856-10915.
44. Topol EJ. Deep Medicine: How Artificial Intelligence Can Make Healthcare Human Again. Basic Books; 2019.”