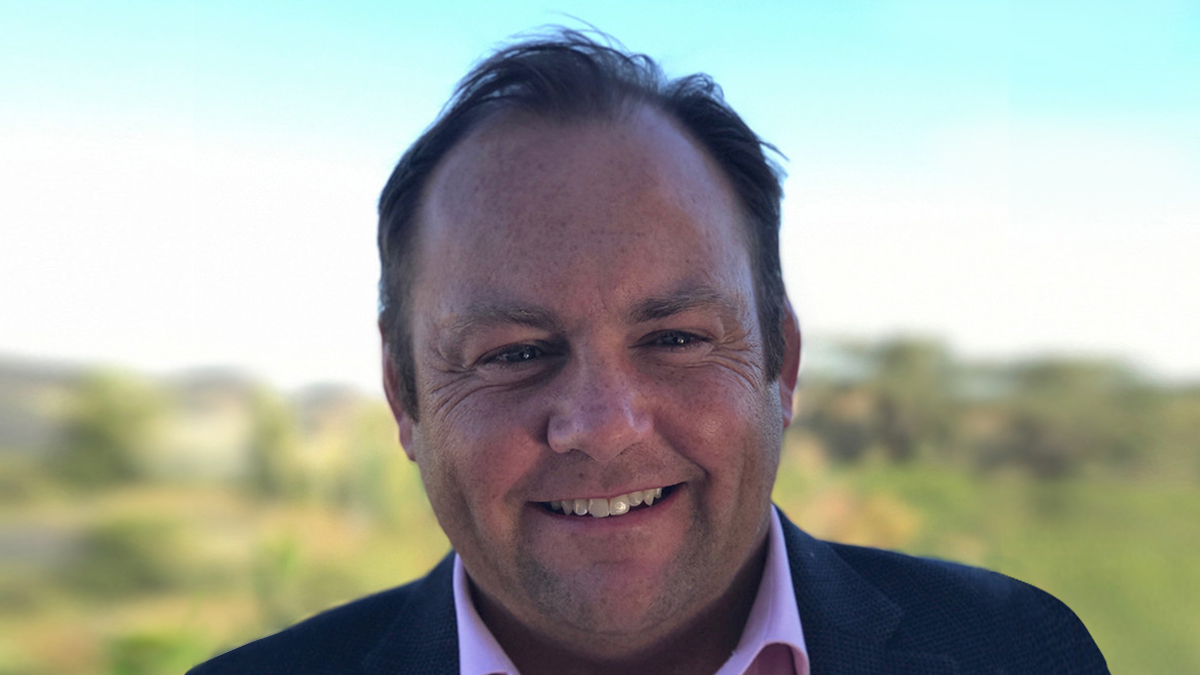
Brian Shields: Pharma’s AI Ventures – Helpful Tips for Senior Executive Leaders
Brian Shields, Founder of KOL Pulse, shared on LinkedIn:
“Pharma’s AI Ventures – Helpful Tips for Senior Executive Leaders
As an Artificial Intelligence developer in the Pharma industry, I’ve had a front row seat to this exciting space. Working directly with database architects, brilliant users, machine learning engineers and full-stack developers has provided me with some interesting insights and some super exciting moments. Sometimes the AI output is like discovering fire!
Below are some takeaways that may help senior execs as they try to map out a future with AI as a competitive advantage:
1. AI user adoption should be your primary goal. Start with pain points and solve these problems with MVPs(minimum viable products). With KOL Pulse AI, we’ve focused on the pain point of content curation in the digital opinion leader space. How can your Medical Affairs personnel gain insights from the tweets, medical conference slides, YouTube videos, and BlueSky conversations by the Digital Opinion Leader community?—without spending all their time on their phones or laptops? KOL Pulse AI
What problem are you trying to solve? If you don’t know, see point #2
Solving Problems = AI User Adoption
2. Data Management: Should you invest in a massive datacenter with all of these wonderful data and create an AI application that will be super smart because it has access to all of these data?
Ummm, how good are your data?
With AI, as Forest Gump told us, Stupid is as Stupid Does!!!
The more data you include in your AI app, the more difficult it will be to achieve your required result. by the way, what is your required result? Do you want simple conference summaries? Do you want predictive insights? What do you want as your output and what data are required to achieve this output?
Size of Data Set = Stupid AI^2
3. How will you test?
This is perhaps the largest pain point in AI application development. As your ML and database engineers work in lockstep to create an AI application that delivers your desired output, do you expect them to understand the fundamental dataset? For example, how will your outsourced ML engineer test a prompt regarding a clinical trial summary with terms such as hazard ratio and PFS? What is an adverse event? What is financial toxicity? How will you test the model? If you outsource it all, don’t be surprised when the outcome is a bit disappointing.
Size of data set = Complexity of testing ^3
4. Hallucinations?!?!?
AI is wonderful, until it creates a piece of fiction that seems legit, and it fools your users. An AI hallucination has the same effect on your users as food poisoning at a restaurant.
How do you engineer the dataset, engineer the prompting, and test for hallucinations within your very specific disease state or treatment category to build an AI app that thrills your users and enhances AI adoption?
Hallucinations = negative user adoption ^5
Tip #5 = Use a proven App like KOL Pulse to build AI Adoption!”
-
Challenging the Status Quo in Colorectal Cancer 2024
December 6-8, 2024
-
ESMO 2024 Congress
September 13-17, 2024
-
ASCO Annual Meeting
May 30 - June 4, 2024
-
Yvonne Award 2024
May 31, 2024
-
OncoThon 2024, Online
Feb. 15, 2024
-
Global Summit on War & Cancer 2023, Online
Dec. 14-16, 2023