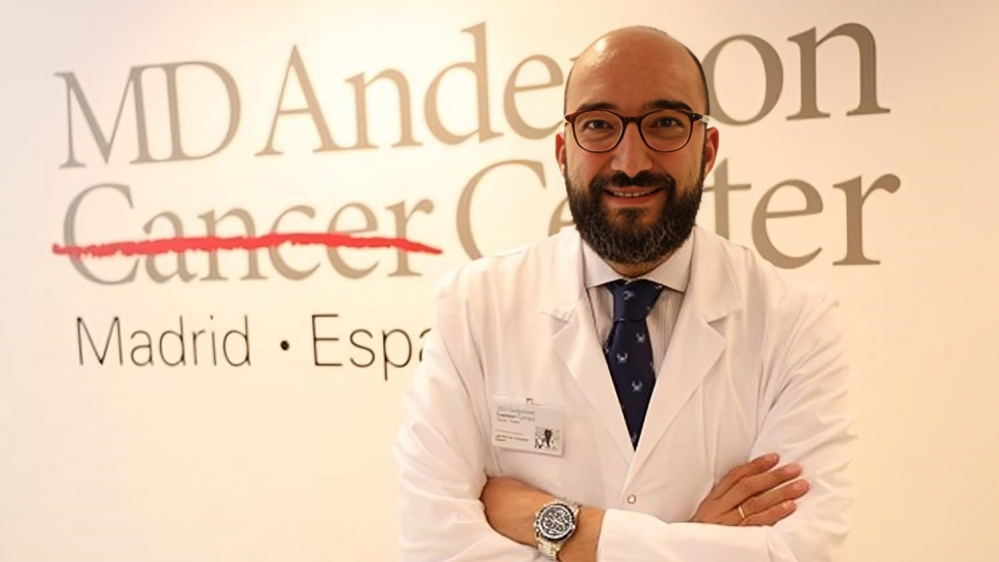
Enrique Grande: Prediction of checkpoint inhibitor immunotherapy efficacy for cancer using routine blood tests and clinical data
Enrique Grande, Head of Oncology at MD Anderson Cancer Center, shared an article led by Seong-Keun Yoo on LinkedIn about predicting the effectiveness of checkpoint inhibitor immunotherapy for cancer using routine blood tests and clinical data.
Authors: Seong-Keun Yoo, Conall W. Fitzgerald, Byuri Angela Cho, Bailey G. Fitzgerald, Catherine Han, Elizabeth S. Koh, Abhinav Pandey, Hannah Sfreddo, Fionnuala Crowley, Michelle Rudshteyn Korostin, Neha Debnath, Yan Leyfman, Cristina Valero, Mark Lee, Joris L. Vos, Andrew Sangho Lee, Karena Zhao, Stanley Lam, Ezekiel Olumuyide, Fengshen Kuo, Eric A. Wilson, Pauline Hamon, Clotilde Hennequin, Miriam Saffern, Diego Chowell
SCORPIO is a machine learning system designed to predict whether cancer patients will benefit from immune checkpoint inhibitors (ICIs) using routine blood tests and clinical data. Trained on data from 9,745 ICI-treated patients across 21 cancer types, SCORPIO outperformed tumor mutational burden (TMB) in predicting overall survival and clinical benefit in multiple internal test sets.
It achieved strong predictive performance for overall survival (AUC values around 0.76) and clinical benefit (AUC values up to 0.71). External validation in global trials and a real-world cohort confirmed SCORPIO’s reliability and adaptability, demonstrating its potential to predict patient outcomes across diverse cancer types and healthcare settings.
Immune checkpoint inhibitors (ICIs) like anti-CTLA-4 and anti-PD-1/PD-L1 agents can lead to durable responses in some cancer patients, but many do not experience long-term benefits despite the high costs of treatment. Current biomarkers like tumor mutational burden (TMB) and PD-L1 expression are FDA-approved but have limitations, such as accuracy issues and practical challenges in clinical use. This highlights the need for a more accessible and reliable predictive model.
This study developed and tested an ML model to predict ICI outcomes using data from real-world cohorts and global clinical trials, potentially improving ICI treatment selection in precision medicine.
-
ESMO 2024 Congress
September 13-17, 2024
-
ASCO Annual Meeting
May 30 - June 4, 2024
-
Yvonne Award 2024
May 31, 2024
-
OncoThon 2024, Online
Feb. 15, 2024
-
Global Summit on War & Cancer 2023, Online
Dec. 14-16, 2023