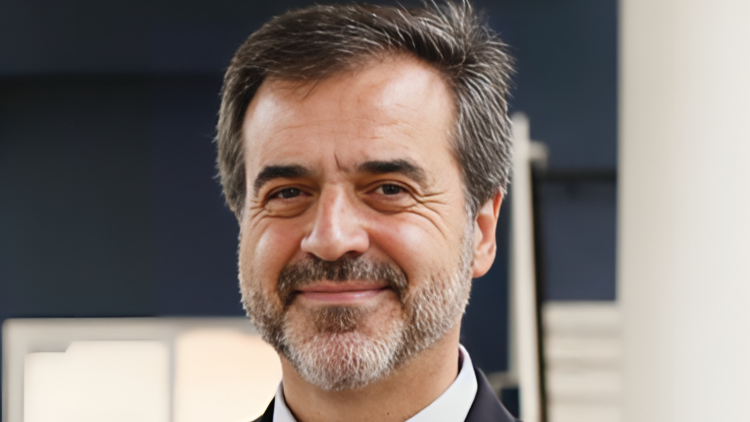
Francisco Esteva: AI reader is making strides in mammographic screening for breast cancer
Francisco Esteva, Chief of Division of Hematology and Medical Oncology at Lenox Hill Hospital, shared a post on LinkedIn about a recent paper by Helen Frazer et al. published in Nature Communications.
Authors: Helen Frazer, Carlos Peña-Solorzano, Chun Fung Kwok, Michael Elliott, Yuanhong Chen, Chong Wang, Jocelyn Lippey, John Hopper, Peter Brotchie, Gustavo Carneiro and Davis McCarthy.
“Artificial Intelligence is making strides in mammographic screening for breast cancer, according to a study in Nature Communications. The AI reader, built on advanced deep-learning models, outperformed or matched 74 out of 81 human radiologists in sensitivity and/or specificity.
The study used both retrospective and prospective analyses, with data from over 25,000 clients and confirmed cancer outcomes through histopathology and cancer registries.
These results suggest that AI can seriously boost the accuracy and efficiency of breast cancer screening. With AI readers matching or beating human radiologists most of the time, adding AI to screening programs could lead to better early detection and outcomes for patients.
However, there are limitations to this type of approach and research methodology. For example, this study did not account for changes in cancer prevalence affecting both AI triage and AI band-pass readers.
It examined human-AI interaction effects in isolation and for a single AI operating point. And it did not consider how human readers might adjust decisions based on AI scores or supporting information.
Future work and considerations:
- Need for prospective studies and dataset development
- Development of ongoing algorithm quality assurance to assess bias and drift
- Further examination of AI explainability and acceptance of AI errors by clients and clinicians
- Improvement of algorithms to focus on interval cancers.”
Source: Francisco Esteva/LinkedIn
-
ESMO 2024 Congress
September 13-17, 2024
-
ASCO Annual Meeting
May 30 - June 4, 2024
-
Yvonne Award 2024
May 31, 2024
-
OncoThon 2024, Online
Feb. 15, 2024
-
Global Summit on War & Cancer 2023, Online
Dec. 14-16, 2023