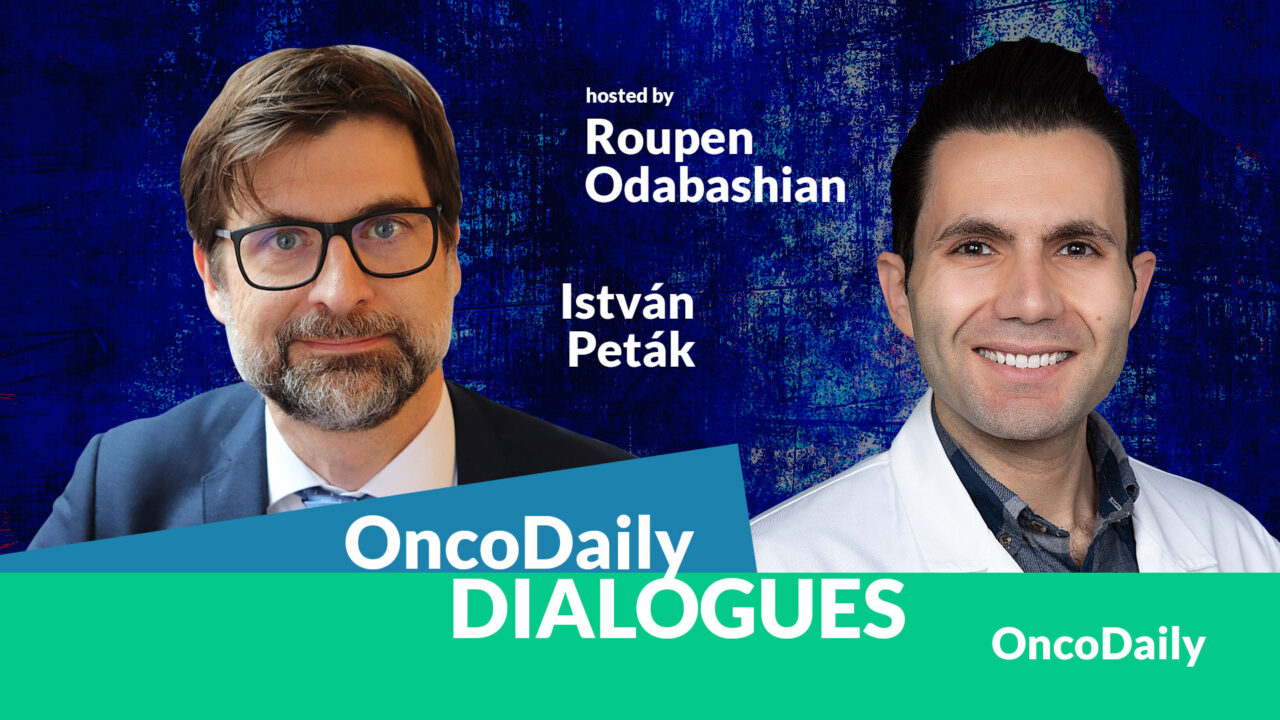
Oncodaily Dialogues #9 István Peták/ Hosted by Roupen Odabashian
In our ongoing series called “OncoDaily Dialogues”, we consistently feature prominent individuals in the field of oncology. We showcase their achievements, obstacles they’ve overcome, and significant moments in their journey.
Today, we’re honored to welcome István Peták, M.D., the founder and the CEO of Genomate health, also the founder and the Board Director of Oncompass Medicine.
In this episode of OncoDaily Dialogues, István Peták discusses Genomate Health’s innovations in precision medicine, highlighting their computational reasoning intelligence system and its applications in oncology. He addresses solutions offered by AI, and shares his personal journey and entrepreneurial insights, providing advice for aspiring entrepreneurs.
About István Peták
Dr. Istvan Petak specialises in precision oncology and molecular pharmacology of targeted therapies. He is the founder and CEO of Genomate Health. Founder and board director of Oncompass Medicine, Budapest, HU, and adjunct professor at the Department of Pharmaceutical Sciences at the University of Illinois at Chicago (UIC).
His main research is exploring the role of apoptotic pathways in individual drug sensitivity, pioneering molecular companion diagnostics of the EGFR gene in 2003, and implementing NGS (next-generation sequencing) in precision oncology in 2008. He is the lead inventor of a novel computational method, for standardized, personalized treatment recommendations that has won numerous recognitions from organizations, including DIGITALEUROPE and ASCO.
About Roupen Odabashian
Dr. Roupen Odabashian is an accomplished Internal Medicine Physician and Hematology/Oncology Fellow with a profound commitment to advancing healthcare through clinical practice, research, and technology. Currently based at the prestigious Karmanos Cancer Institute, Dr. Odabashian is actively involved in pioneering cancer treatments and conducting clinical research.
In addition to his clinical work, Dr. Odabashian is a multifaceted healthcare professional. He hosts podcast at OncoDaily, engaging with leading experts in oncology to share valuable insights with the medical community. Dr. Odabashian also contributes his expertise as an advisor at Spiraldot Health and Mesh AI, supporting innovative ventures in healthcare technology and collaborative scheduling to combat clinician burnout. With his diverse roles and unwavering dedication, Dr. Odabashian exemplifies a commitment to driving positive change in healthcare.
István Peták on Precision Medicine: Challenges, Journey, and Future
00:00 Introduction
01:29 About Genomate Health
02:47 Clinically Validated Computational Reasoning Intelligence System of Genomate
08:06 Precision Medicine Challenges
12:27 Precision Medicine Challenges and AI Solutions
19:08 Discussing the 2021 “Nature” Paper on Precision Oncology
21:35 Cancer Heterogeneity and Precision Medicine Solutions
29:24 Using Genomate Software to Increase Progression-Free Survival
35:04 Dr. Peták’s Personal Journey in Oncology and Precision Medicine
41:38 Entrepreneurship, Scientific Research, and Precision Medicine
46:52 Recommended Books and Advice on Becoming an Entrepreneur
48:48 About Discipline and Focusing on Problems
The Transcript of István Peták on Precision Medicine: Challenges, Journey, and Future
Roupen Odabashian: Hello everyone and welcome to a new episode of OncoDaily. I’m very, very, very excited today. I have a very special guest that I’ve been waiting for this interview for a while. You will learn a lot and think this is the dream of, for me at least and many physicians who want to make a difference, by creating companies and making real difference, especially in the field of oncology.
So, my guest today is Dr. István Peták. He’s an international expert in precision oncology and molecular pharmacology of targeted therapies. He has over 25 years of experience. He’s the founder and the CEO of GenoMate Health and he’s the founder and the board director of Oncompass Medicine. He’s an adjunct professor at the Department of Pharmaceutical Sciences at the University of Illinois at Chicago.
He’s the lead inventor of a novel computational method published in 2021 for standardized personalized treatment recommendations that has won numerous recognitions from organizations including Digital Europe and ASCO.
Dr. Peták, thank you so much for being here today.
István Peták: Thank you so much for having me. It’s a pleasure.
Roupen Odabashian: Likewise. I want to start talking about one of your babies which you were talking about earlier, GenoMate. So can you explain to the audience what does GenoMate do?
István Peták: Sure. So, if we want to summarize it in a sentence, this is a clinically validated computational reasoning intelligence system. That means that, we can take, a complex molecular profile of a cancer sample from a cancer patient, compute it, and match, this complex molecular profile to treatment recommendations using, a computational model, a computational method.
We can use, call it artificial intelligence, but the point is that, we created a mathematical model we have been able to validate that really maximizes the chance that we make the right decision, when we try to find the most likely effective molecularly targeted therapy for our cancer patients.
Roupen Odabashian: Got you. And, can we talk through, like, the steps? Like, let’s say I’m an oncologist in the clinic and I, I want to order a report, or I’m a patient. So what are the steps to get to the results that GenoMate provides?
István Peták: Sure. Uh, so very practically, if the patient already has a molecular diagnostic test ready, typically including at least one, next-generation sequencing-based molecular profiling test, there are many, many vendors. Just I, I can, I can, name a few: Foundation Medicine, or Tempus, or Caris. So these companies create great molecular diagnostic tests and they test hundreds of genes at the same time.
And so, typically they identify an average of four to five driver alterations, driver genetic alterations per patient, and they create a report on these alterations. and also the same patient may have other tests, single gene tests using PCR or FISH or immunohistochemistry. So the same patient can have also PD-L1 immunohistochemistry results and, and maybe the same patient will have multiple other test results, even NGS like maybe another, a liquid biopsy-based test as well, from Guardant, for example.
And then, so you, you, you sit in front of your patient and you have all, all these reports with, and each will have a different, list of alterations. And in these reports, are created by softwares using softwares that use knowledge bases to actually interpret these genetic alterations.
So, you know, so you have a, in these reports, you also see which is a, a pathogenic driver, what is a, which one is a variant of unknown significance, and, and, and if there is a matching therapy or you also see, uh, the therapy that is matched. Now the problem is that of course, these reports link these genetic alterations to different therapies separately, one by one.
So one alteration to one treatment option usually and, and the level of the connection is based on, the, the reference the, the scientist found who built the knowledge base. And so, and we know this ASCO and other recommended ways to tier these connections.
Sure, the problem is that it’s extremely confusing when you have multiple treatment alterations and multiple tests, but as an oncologist, you have to make your final decision based on the totality of the molecular information you know about the patient. Each gene or genetic alteration can be linked to multiple treatment options, and each treatment option can be linked to multiple genetic alterations in the same patient. So, how can you make the final decision?
All these test results are the inputs of our system. You can upload the reports to a secure HIPAA-compliant portal, and we send you back the GenoMate report. You can also log in to our interactive portal where you can explore the thinking process of the computational method. It will rank treatment options based on a score called the GenoMate score. It’s an actual mathematical number calculated by the system.
Each treatment option has a score, scientifically called the aggregated evidence level, or AEL. The whole model is called the Digital Drug Assignment System. The whole logic is that you have a very easy-to-understand objective ranking of treatment options that are assigned to the totality of the molecular profile, the whole molecular profile. What is the final inference, the final conclusion for this patient based on all the alterations?
So, in a nutshell, this is how you can already use it. We have already set up pilots and collaborations, but our ultimate vision is to create a companion diagnostic software platform out of this and really make this dream come true. We, physicians, oncologists, and healthcare providers, can provide this n-of-one precision therapy we all dream about. We talk about this a lot, but the difficulty is that without a validated new type of medical device that has been validated to provide the right n-of-one therapy, we cannot really practice precision medicine in reality.
When we talk about precision oncology or precision medicine, we usually think about therapies that have a companion diagnostic test, a molecular diagnostic test approved as a companion diagnostic predictive test. But that is not precision oncology because that is just a biomarker that has been used to select patients through a clinical trial, proving that patients who carry this biomarker have a higher statistical chance to respond to that targeted therapy.
The difficulty is that the same patient will have, on average, four other biomarkers as well. We conduct these clinical trials by selecting inclusion criteria to try to make a homogeneous population of patients and then try different drugs in this population. But in oncology, in precision oncology, we know that on the molecular level, cancer is so heterogeneous that actually the number of molecular subgroups is enormous. Even if patients share the same biomarker, you don’t really know if your patient should benefit the most from a therapy against one or the other biomarker in his tumor.
I give you a very simple example that is widely known by oncologists. When you have a breast cancer patient who is BRCA mutant and it’s metastatic, you know that there are PARP inhibitors approved. Absolutely, and we also know that HER2-positive, overexpressing breast cancers benefit from HER2 inhibitors. We have multiple options now. So what happens if you are presented with a patient who is BRCA mutant and HER2 positive? It’s rare, but it happens.
Roupen Odabashian: It happens, yeah.
István Peták: It happens. Then we need a randomized clinical trial to compare HER2 inhibitors and PARP inhibitors in this population
Roupen Odabashian: Which is very hard to do because those are already rare patients.
István Peták: Already rare. And on top of that, that’s just one current case. A case we had, of course, had other mutations or alterations. The patient will have another two, three, or four other alterations like MYC amplification. So, do we know what to do with this information?
Does MYC amplification increase or decrease the chance to respond to PARP inhibitors or HER2 inhibitors? If we knew that MYC amplification causes resistance to one or the other, to the PARP inhibitor or the HER2 inhibitor, it would be easy to make the decision.
Okay, even if this is a HER2-positive cancer, we would use the PARP inhibitor first for this case. But of course, there’s a clinical trial for patients with MYC amplification. But should I send my patient to this trial because I should know that maybe the HER2 amplification causes resistance to MYC inhibitors?
So, actually, at the end of the day, we theoretically need evidence for each molecular subtype for each combination of mutations. We have only 700 cancer genes, around 700 according to COSMIC. That doesn’t sound like a lot. It’s not really scary. We have 25,000 genes, and in the past 20 years, we conducted this systematically with the Cancer Genome Atlas Project. In 2020, there was a great paper from this huge consortium of whole genome sequencing of thousands of tumors.
They reported that now we can identify the genetic cause of cancer in 95% of cancer patients. So, actually, we can find a driver, at least one driver, in 95% of cancers. The good news is that it’s a closed box. There are a countable number of genes, a countable number of cancer genes.
The problem with the 700 genes is that it’s more than 100. If it’s more than 100, the average frequency is less than 1%. On top of that, you have the different types of mutations in the same genes. Then, on top of it, you have the combinations.
Roupen Odabashian: Exactly. I completely agree with that. It’s like 700 to the power of I don’t know which number because you can come up with many different combinations. I think this is a problem that we all run into. You send one tissue molecular test, and it might come back negative or positive. Then you send the circulating tumor DNA, and it might come back discordant, showing another mutation.
Then you have to decide what to do now, which one should guide my treatment. Like the example that you gave, it’s something that we run into. I have those two mutations. How do they interact? What should I use? Should I use drug X or drug Y? These are all valid problems that we go through in the clinic as oncologists, trying to practice precision medicine. It is very frustrating.
I’m happy that you are creating a solution that can consolidate all the results because I also believe that, as human beings, we can’t really retain and process the amount of information that is being released every day when it comes to precision medicine—the different genes, the different treatments available. It’s crazy. It’s beyond our capacity, and that’s why we create things that help us, like GenoMate.
István Peták: Absolutely. I think we just have to embrace a new generation of medical devices, software as medical devices. The good news is that patients seem to like this. The idea that the oncologist uses an AI to augment his ability or chance to make the right decision or uses different type of software in different type of situations, it just sounds very logical.
So ultimately, what I think what the evolution of medicine dictates is that after decades of research to understand the biology of the human body, to understand the biology of cancer and having 30 plus million PubMed articles, and also our ability to actually measure biomedical parameters and do whole genome sequencing for a thousand dollars or less.
So this we generate all this data and we are now digitalizing everything. And so we have a lot of parameters as an input and we have multiple treatment options. That’s the good news, that we have over 200 targeted therapies approved by FDA to choose from.
Roupen Odabashian: Yeah. Yeah. Our toolbox is huge now.
István Peták: The input and the output possibilities are great. And so the number of variations, I mean, possibilities is exponential. So the problem is the exponential nature of this.
So when I graduated almost 30 years ago and I went to medical conferences first, we had like a dozen tumor type and a dozen chemotherapies. So we compared all the chemotherapies to each other in randomized clinical trials in all tumor types. Yeah.
So but when years came by and I saw that, oh, now we have 20 drugs and we subcategorized tumors based on immunohistochemistry. And now we have 100 tumor types and we have 100 therapies. Exactly.
Roupen Odabashian: It’s mind blowing. Every cancer, and that’s what I tell all my patients, like every cancer is an orphan disease. Like no cancer is like the other.
And you have to know what makes each cancer and each patient different and unique. Like none of the cancers are similar to each other. I want to shift gears a bit and talk about your paper, which is like, it’s very interesting.
So in 2021, 2022, you published a paper in Nature about computational method for prioritizing targeted therapy in precision oncology and performance analysis in the SHIVA-01 trial. So since now we understand how Genomate works, I want to go to your paper and tell me a bit about what type of study was it and what were the main results.
If physicians are using computational method to choose the right treatment, what are the outcomes when it comes to the PFS-OS that we could expect when using software to guide our treatment?
István Peták: So we are very proud of this paper because I think this is the first paper, according to my knowledge, that proves that using a computational method, we can improve our chances to use the right personalized therapy when we try to implement the concept of precision oncology.
So the data we use was created by Christophe Tourneau and his team at the Institut Curie in Paris, and he was really a pioneer of precision oncology. And this SHIVA-01 was the first randomized clinical trial in precision oncology. So basically, their molecular tumor board selected drugs based on the molecular profile of the patients out of 11 drugs they had.
So they predefined biomarkers as an inclusion criteria for them. So genetically, all patients who were included in this clinical trial were treated with a matching therapy. So because that was the inclusion criteria to have an actionable biomarker.
A mutation, yeah. So what they could achieve is a 56% overall response rate, which you can, it’s a bottle of water half full.
Roupen Odabashian: Exactly, but also like taking into account that you have different heterogeneity in cancer types and their targeted therapies too.
István Peták: So this was a solid, it was, of course, very early stage patients after multiple lines of therapies and different solid tumors.
And so what, so I just really tell you the exciting part that when we reanalyze this molecular profiles of these patients and ask our model, a computation model to predict whether the therapy the patient received would work or not, we could identify patients who had a much higher chance to benefit from these matching therapies and those who did not benefit.
So patients who received the targeted therapy that was also supported by the model had like 69% response rate, and those who were predicted to be non-effective is for 17%.
Now, the difference is fourfold. And also it’s exciting because on the other arm of this clinical trial, the average response rate to chemotherapies chosen by the oncologists were also around 50%. So basically, this computation model could have helped these oncologists already to predict whether the patient would benefit more from just an off-label treatment based on the presence of the molecular profile or from chemotherapy.
So, and so I think, and the, of course, the reason why the model was better is because the model took into account all the mutations for each patient. So even if, so it’s, so because the whole concept that you make your decision based on the presence of a single biomarker, a single gene. So, so the pre, our hobby practice, precision oncology, you think, okay, I’m looking for patients with PI3K mutations because I have a PI3K inhibitor.
And I, I don’t care if the same patient has also KRAS mutation, HDR2 amplification, whatever. I just look for patients with the PI3K mutations. I mean.
Roupen Odabashian: That’s right. That’s what we do sometimes, or most of the times in the clinic.
István Peták: But you should not be surprised that you have a very heterogeneous response to your targeted therapy.
If you base your decision on the presence of just one gene, although you know that each patient will have three, four, five, six other mutations. So that’s the, that’s the difficulty. So, so when we practice precision oncology in a sense where we want to really choose a therapy based on the molecular profile of the patient, we, we must be able to choose which gene to target.
We must be predict, if you have five genetic alteration in your condition medicine report, and you have three drugs, drugs for three out of the five, you have to know which, if any of the three drugs will be effective as a monotherapy, even in the presence of the other four.
Or you need a combination therapy, if you need a combination therapy, which therapy to combine, or you have to just, you just, you have to make the decision that you must use a chemotherapy, because today we don’t have the right targeted therapy for this patient, and the patient would benefit from chemotherapy more.
So, and, and the whole concept of precision oncology, what is so beautiful about it, is that we, we think that theoretically we should be able to reason this out, because the whole concept of precision oncology is that we understand the biological significance function of these cancer genes, and we know the molecular mechanism of action of our targeted therapies.
So, because by definition, we know the targets of the targeted therapies. So, so the whole concept of precision oncology, that’s why I became fond of this and in love that we could use our common sense to treat these patients, and, and the, and the difficulty is, is just, is the, is the number, is the number, the level of complexes, it’s a mathematical problem.
And that’s so exciting that now we can detect all the driver mutations in our patients, because we have the tools, and we have, and now, so what, what I really hope is, is that with solutions like ours, we can really practice precision oncology in our everyday clinical practice.
And for our patients, and also what I really hope to accelerate the clinical development of drugs, because we will be able to select patients to clinical trials that not only are likely to benefit, but patients who most likely benefit the most from that therapy compared to other treatment options.
Because that’s the difficulty, is to find the niche of each drugs to know which are the patients who are benefiting more from this drug than another drug, without doing the, all the possible randomized trials that we cannot do for all these subtypes. And so ultimately, the vision is that we will have this companion diagnostic AI guided drug assignment algorithms, and then we will know in which indication we should use which one.
Because there will be clinical trials, because we already have this clinical trial data published, but we will, sure, we want to much more, and we also embrace a future where others will have other solutions similar to ours. And I’m just really looking forward to have randomized clinical trials again, but not again comparing drugs, but to compare. Comparing softwares? Comparing softwares.
That would be very, very cool. We will have trials again, but just comparing drug assignment algorithms to each other. So you want to know in how much percent of patients the software chooses the right therapy in one arm and the other arm.
And this is what also patients want to know, by the way. They are not interested in the clinical trial data of the drug, how much percent of patients responded to this drug you are recommending to the patient. They want to really know is your ability as an oncologist to choose the right drug for them.
Roupen Odabashian: Yeah, exactly. One hundred percent, I agree. Like, no one cares about the average.
Like, 50 percent of the patients responded. Well, does that mean, like, what are my chances? 50-50. It’s just like flipping a coin, right? Am I going to be the 50 that will respond to the treatment, or am I going to be the 50 that will not respond? And what we tell patients, we don’t know.
And that’s the frustrating part. We just, like, throw this drug. It might work or it might not work.
It’s frustrating from both ends, like the patient and the physician, and I share your frustration. So if I’m going to summarize, I have the trial open here in front of me, and I went through it several times.
For people who want to read the trial, we’re going to leave the link in the comments. If I’m going to make it simple, I would say using GenoMate software to choose the right drug for patients increases progression-free survival duration. Is that right?
István Peták: Yes, even so, we could prove a statistically significant difference in terms of progression-free survival in this trial. Although the absolute numbers were just a few months, even that was significant. Despite the low number, it was like hundred and 12 patients, but still, the difference was so big that it became statistically significant. Of course, that was the first paper we published on this, and I really want to embrace it because it shows that the concept works. We know that precision oncology works.
So, I was in the team and I’m very proud of a lung cancer patient who had EGFR mutations. We organized a single-patient clinical trial for this patient based on the presence of the activating mutations in the epidermal growth factor receptor before there was any publication suggesting that there is a link. So that was just pure intuition.
I mean, not rocket science. There was a lung cancer EGFR mutation, and also it was helpful that she also had an amplification of the gene. So when you see 100 copies of a mutant allele, that’s very suspicious. And then your reasoning is just that, okay, maybe I should treat this patient with an inhibitor of this growth factor receptor who has been amplified 100 times in this patient.
I mean, it’s like, usually the example I say is that when you see, as an example, that imagine you are in an ER and there’s a patient who comes to you complaining about a headache. You go closer and you see a big nail in his head. So what would you do? And then, of course, I always joke that, of course, you treat for migraine because that’s the most common cause of headache. That’s evidence-based. But now, in oncology, we see the nails before..
Roupen Odabashian: Exactly. I agree that was a good example.
István Peták: Then we don’t want to ignore that. That patient had a complete response and lived tumor-free for five years. This was a transforming event in my life and career when I saw the power of precision oncology or using the right targeted therapy that can really eliminate the multiple metastatic sites in a patient.
At that time, we were very skeptical because cancer is a very complicated disease, heterogeneous, multiclonal, and all that, with multiple sites. But using just a targeted therapy, the whole system can collapse if it’s the right one. We know that the concept works. We know that many patients respond very well. The frustration is that we cannot expand this to more patients.
The reason is simply that it’s just a bit more complicated. Patients come in, they have a lot of nails. Many are passenger nails, so they are there but they don’t cause headaches or anything. And then you have four or five nails with different impacts, which actually contribute to the headache.
You have to make a decision in the ER quickly about which nail to pull out first. Now it’s getting complicated. But, of course, we have such a detailed knowledge of the anatomy of the brain. We know where the nerves are going and how they are affected. We can do CT scans and see how deep the nails go in and which one touches which nerve. Sure, I mean it’s an imaginary example…
Roupen Odabashian: But this is a great analogy. This is exactly what we deal with in the clinic, right? Multiple mutations, multiple discrepancies between tissue testing and blood testing, and then good luck choosing the right one.
It’s very hard. For the sake of time, I want to shift gears and talk more about you. I’m very impressed by GenoMate, but I believe you also have so much value to teach the younger generation of oncologists about where you are and where you have been. Can you talk to me through your early residency and training days? What made you pursue a career in oncology and then go to precision medicine?
István Peták: Sure. If I really want to go back, sorry for just very briefly, even to my childhood, when I first received a tortoise from my parents. I had to take care of that animal, and I didn’t know what to do. I went to the library and borrowed a book about how to keep animals like tortoises.
I learned that it was a Testudo hermanni boettgeri in Latin, and I learned what kind of food the tortoise likes. From that, I really became fond of this idea that by just knowing things, we can save lives. Understanding how nature works, we can save lives.
When I graduated from medical school, I went into pediatric oncology first. That was the ultimate challenge, I think, for a doctor to work with young children with cancer. At that time, one of my tasks was to treat patients with acute lymphoblastic leukemia (ALL). In 1995, we already knew that 5% of ALL patients actually harbor the Philadelphia chromosome, the BCR-ABL translocation.
Those patients had a worse prognosis and didn’t respond well to chemo. It was frustrating that we had to give the same chemotherapy protocol, knowing that they would die.
I took blood from these patients and took it to the molecular pathology department, isolated the cells, and tried to find ways to kill these cells. That was the dawn of apoptosis research. We started to understand that the life and death of cells are molecularly regulated so it’s an active response to these therapies.
We also knew at that time that the BCR-ABL fusion protein is the actual cause of cancer in these patients. If you put this fusion gene into a mouse, the mouse develops leukemia. In vitro, if you use antisense oligonucleotides to silence the gene, these leukemic cells stop growing. This is the nail.
In 1995, we didn’t have the tool to pull it out, so we had to wait until 2000 when imatinib was approved. It was a breakthrough, and the good news is that not just for CML patients but also for these children with ALL, they can now receive imatinib and survive.
I was really lucky. I went into research in molecular pharmacology and studied signal transduction pathways to understand how cell growth and cell death are regulated and to choose the right targets. That’s when I went to St. Jude Children’s Research Hospital for five years. I did research there and published papers on apoptotic pathways.
It was exciting to see the big differences in drug sensitivity between cells. It was obvious that it would be the same in practice if you want to use targeted therapies. It’s not like chemotherapy, which is dose-dependent. For targeted therapies, what you see is that they either work or they don’t. It was obvious that we must have the tools to detect these genetic alterations and to predict whether that particular patient will respond to our therapy or not. Otherwise, we will not be able to use these targeted therapies.
This is why in 2003, I decided to start my first company with my classmate from Semmelweis University, 21 years ago. We started to develop the first molecular diagnostic tests for EGFR. Fifteen years ago, we started NGS in 2008. When we started to see that patients have not just one alteration, just one nail, but multiple, we realized that we needed a third innovation that’s now also by an AI fold and other fantastic innovations.
Now we have the ability to detect these mutations and develop molecularly targeted therapies. We need a third class of tools: treatment selection computational tools or AI-guided drug assignment algorithms that actually match these complex molecular profiles to the right targeted therapy.
Then we have everything: the three classes of tools, like in Harry Potter, to conquer death and conquer cancer.
Roupen Odabashian: I want to go back a bit to the early days when you started transitioning from clinician to entrepreneur. How was it? How did you know what to do? When you say that you started a next-generation sequencing company, there’s a lot to it. It’s not easy. It’s a full-time job requiring fundraising, bringing in machines, and building teams. How were those early days, and how did you learn to start a company?
István Peták: First of all, I had to make the decision that I wanted to create tools other doctors could use. Although I love working with patients, I thought my mission was to develop new technologies for other doctors to be more successful. That was the first decision. Another was when we discovered the first cancer genes and understood the biology of cancer. I saw how difficult it was to translate this knowledge into clinical practice.
It was just unbearable for me that we already knew the solution, it was published, and it was not used in clinical practice. I understood that it’s a very complicated multi-step process from scientific discovery until we can use a new innovation in clinical practice, whether it’s a diagnostic method or a therapy.
The bridge is an innovation-driven enterprise or biotech company. Between basic research and practice, actually, 80% of drugs we use today are developed by small biotech companies. Large pharma companies buy these little companies; they acquire them. But there is an innovation pipeline and an important role for these small biotech companies that translate research into a practical tool, a medical device, or a drug.
I’m so excited and really enjoy working on how to accelerate the translation of scientific discoveries into clinically useful tools and so that’s why I became an entrepreneur and the I think scientific research is really close to entrepreneurship is because it’s about Doing something new to address an unmet medical need, or any need really, with a completely new approach is quite challenging.
You don’t really know if it’s going to work or not, and it can be extremely stressful. But on the other hand, it’s also very exciting. So, I think entrepreneurship isn’t that far removed from research and discovery, or even clinical research.
Of course, there are a lot of business aspects I had to learn, but I have a team that supports me. I also love the opportunity to build a team and create a new entity from nothing, a new society of humans working together synergistically to create something we couldn’t achieve individually. It’s beautiful, and I really enjoy this part. But of course it’s exhausting to balance fundraising, research, and leading the team.
Being part of the story where we see patients responding better to therapies thanks to the tools we created is incredibly rewarding.
Roupen Odabashian: I completely agree with you. The point of research is very similar to entrepreneurship because you have to build a team, come up with ideas, develop methods, and secure funding. It might seem far apart, but it really isn’t. It is different in its different ways. You mentioned learning about the business side, which isn’t something we learn in medical school.
Do you have any recommended books or courses for someone with an idea who wants to start building something big like you did? What they should read. What they should learn?
István Peták: I didn’t have any formal business training, no MBA. We started our startup 20 years ago, just two of us with my friend and gradually grew. I learned by doing. I think, if you want to be an entrepreneur with an MD or PhD background, you need the right advisers and team. Ideally, your co-founder can have a business background. Finding angel investors who can also be advisers is crucial before approaching venture capitalists.
There are many courses and books out there. I’ve been inspired by books like “Zero to One” by Peter Thiel and the life of Steve Jobs. Another great book i got from MIT course is “Disciplined Entrepreneurship” from an MIT course, which is very pragmatic. The title also
Roupen Odabashian: I completely agree. One of the biggest challenges in entrepreneurship is how to stay disciplined and focus on one idea.
Yeah, no, I completely agree with you. I think one of the biggest challenges entrepreneurs face is how to stay disciplined. You have tons of ideas, but how do you stick to one and systematically build your strategy?
István Peták: Yes, exactly. You have to focus on the problem you want to solve. There’s a saying I love: you must be in love with the problem you want to solve, not with the solution. I decided from the beginning that I would focus on a problem and develop the tools to achieve my goal.
I decided, thirty years ago, as a physician scientist to focus on a single problem: precision oncology. The aim was to match the right targeted therapy to every cancer patient based on the individual molecular profile of their cancer. This nail problem so why don’t we cannot just really treat every patient based on the understanding of the cancer genetics.
So we should be able and why we don’t and at the beginning I so that was always the goal and then at the beginning I was focusing on technologies that enabled us to detect this IM mutations. And then I switched to computational methods when I realized that this is what we need to get closer to our dream.
So and when we and this also in general it’s there this is what they say, that when you develop a product you need Market feedback and we have to be ready to change and pivot you so you always pivot your strategy, you don’t pivot.
That’s the only, but you focus on the the goal where you want to get to the if it’s Himalaya in the Mount Everest or whatever you just want to get to the top, but on all your way you go around the gers and rock falls and and then you think that you know the path but it’s a dead end so have to start again, so you have to be ready to pivot and adapt but never never change your goal.
Because if you have too many goals, if so, you have to find, there’s another saying I love, ‘you have to find your and choose your battle’ exactly you can’t go into every war, cannot win all the battles of the world but you can choose one battle if you fight long enough but you have to be ready to use whatever tools you need to achieve this goal. So I think that’s in not what I learned in my past decades.
Roupen Odabashian: Awesome, great, thank you so much Dr. Petak, that was very-very-very insightful and we will make sure to leave the link of the paper and other resources, like the book you mentioned, maybe in the comments below so people can read. And the paper it’s very interesting and it is very also like I’m very optimistic about the future of precision medicine, especially when we bring softwares and computers to help oncologist to make decision. Thank you so much for being here today.
István Peták: Thank you so very much for inviting me, thank you, thank you.
-
Challenging the Status Quo in Colorectal Cancer 2024
December 6-8, 2024
-
ESMO 2024 Congress
September 13-17, 2024
-
ASCO Annual Meeting
May 30 - June 4, 2024
-
Yvonne Award 2024
May 31, 2024
-
OncoThon 2024, Online
Feb. 15, 2024
-
Global Summit on War & Cancer 2023, Online
Dec. 14-16, 2023