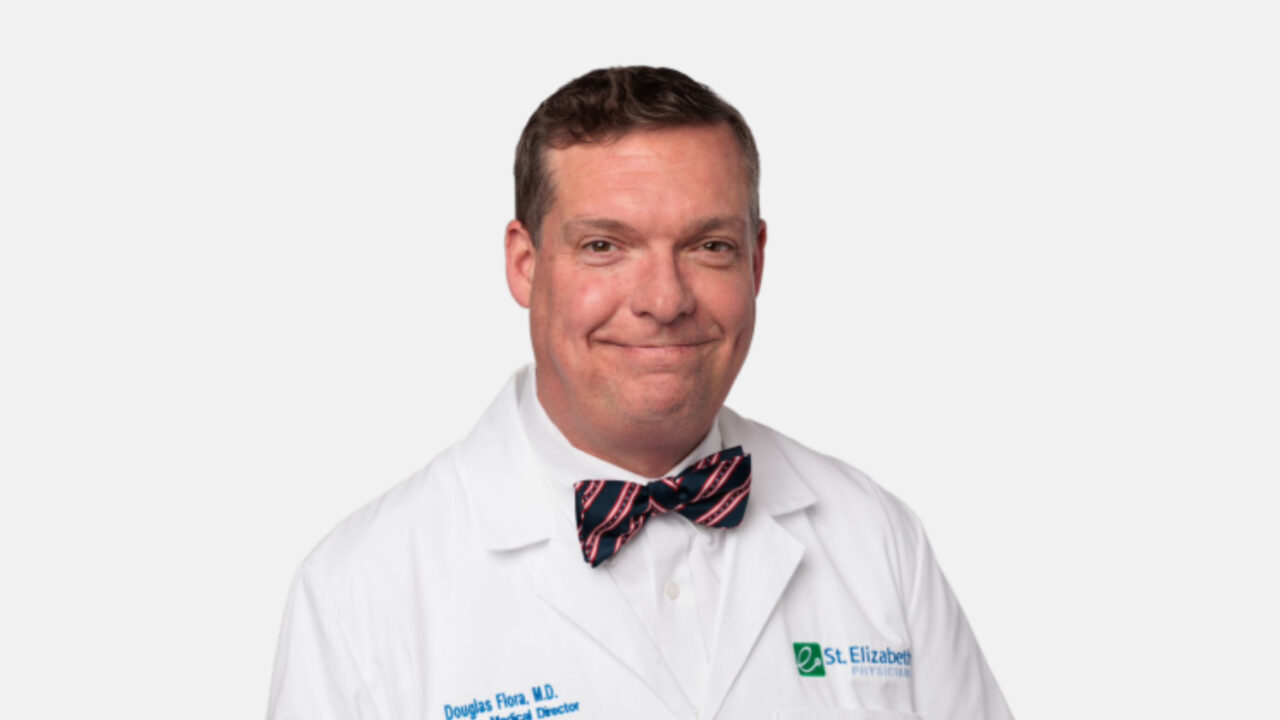
Douglas Flora: Intercepting Malignancy – How AI is Enabling Earlier Cancer Detection
Douglas Flora, President-Elect at Association of Cancer Care Centers, shared a post on LinkedIn:
“The history of medicine is, in many ways, a history of learning to see. From the earliest clinicians relying on the direct evidence of their senses to decipher the body’s narratives through touch and sound, to the transformative moment when lenses first bent light to reveal the hidden world of microbes and cells, progress has often hinged on acquiring new ways of perceiving. The late 19th century brought another such revolution with Röntgen’s discovery of X-rays – invisible emanations that could pierce flesh and etch the shadows of bones onto photographic plates, offering the first non-invasive glimpse into the living human interior. This newfound sight, however primitive initially, fundamentally altered our relationship with disease. Yet, each new way of seeing, from the oil immersion lens that brought bacteria into sharp focus to the sophisticated scanners that map metabolic activity today, eventually encounters its limits – the boundaries of human interpretation, the constraints of fatigue, the sheer overwhelming complexity of biological information.
Now, we stand at the threshold of another profound perceptual shift, one driven by the analytical power of artificial intelligence. As we confront cancer, the multifaceted adversary arising from the intricate code of our biology, AI is offering us tools that not only enhance our existing senses but also provide fundamentally different ways of perceiving malignancy. It’s akin to gaining not just a more powerful microscope, but one capable of seeing patterns in light and shadow, in cellular arrangements, and in molecular whispers that were previously beyond our ken. This is not merely a technological advancement; it is a potential reboot of our entire approach to cancer detection, aiming to intercept the disease not when it looms large, but when it takes its first, faintest footsteps.
Illuminating Shadows, Perceiving Textures: AI in Radiology
The radiologist’s reading room, bathed in the cool glow of monitors, remains a vital setting for detecting cancer. Decades of innovation have filled these screens with increasingly detailed anatomical landscapes captured through CT, MRI, and other modalities. The human expert navigates these complex visual terrains, searching for the subtle anomalies—a density, a distortion, an unexpected enhancement—that herald malignancy. This interpretive skill is remarkable, honed through years of recognizing visual gestalt and correlating patterns with pathology.
Yet, the sheer volume of data in modern imaging studies—thousands of slices, millions of pixels per study—challenges the limits of human capacity. Furthermore, human perception excels at recognizing defined shapes and structures but may struggle with quantifying subtle, distributed patterns or maintaining perfect consistency over thousands of interpretations. A vast realm of potential information exists within image pixels—subtle textural variations in apparently normal tissue surrounding a lesion, complex measures of shape and boundary irregularity, and intricate patterns of vascularity—that may hold diagnostic or prognostic clues but are difficult for the human eye to assess or quantify reliably. This is the domain of radiomics, where computational methods extract vast arrays of quantitative features from images, seeking signals beyond human visual interpretation.
Artificial intelligence, particularly deep learning, thrives in this high-dimensional space. Trained on enormous datasets of annotated images, AI systems learn to identify complex, multi-scale patterns correlated with malignancy. They don’t just look for ‘lesions’; they analyze the entire context, quantifying textural heterogeneity, assessing fractal dimensions of boundaries, and potentially identifying subtle systemic effects reflected far from the primary site. They can compare current scans to priors with meticulous precision, detecting minute interval changes that might escape notice. Studies exploring the use of AI in analyzing screening mammograms and low-dose CT scans for lung cancer suggest that these systems can achieve high levels of accuracy, sometimes flagging suspicious findings that were missed during initial human review or helping to differentiate between benign and malignant lesions with greater confidence.
This AI-driven perception is fundamentally different. It doesn’t rely solely on the learned visual heuristics of human experts but builds its internal representations of disease patterns directly from the pixel data. It can operate tirelessly, consistently applying its learned criteria across vast numbers of studies. This doesn’t obviate the need for human radiologists; instead, it sets the stage for a powerful synergy. AI can function as an indefatigable pre-reader, highlighting potential areas of concern, performing complex quantitative analyses, and bringing patterns beyond human perceptual thresholds to the attention of the expert. The radiologist then integrates these findings with clinical context, assesses plausibility, manages ambiguity, and ultimately makes the diagnostic judgment, their expertise amplified rather than replaced. While rigorous validation and seamless workflow integration remain critical challenges, AI is undeniably providing radiology with powerful new eyes.
Decoding the Cellular Tapestry: AI in Pathology
Pathology remains the bedrock of cancer diagnosis, the court of final appeal where tissue itself reveals its secrets under the microscope. Building on the 19th-century foundations laid by Virchow, pathologists interpret the intricate language of cells—their size, shape, staining characteristics, arrangement, and interactions—to classify tumors, determine their grade (a measure of aggressiveness), and assess their extent. This detailed morphological analysis, performed on meticulously prepared and stained tissue sections, guides critical treatment decisions.
However, this essential practice faces growing challenges. The sheer volume of slides generated by biopsies and surgeries strains capacity. Increasingly complex classification systems demand integration of subtle morphological features with molecular data. And despite rigorous training, an element of subjectivity persists in interpreting complex patterns, resulting in inter-observer variability, particularly in cases with borderline outcomes or assessments.
Digital pathology, which transforms glass slides into high-resolution digital images, created the necessary substrate for AI’s intervention. Now, deep learning algorithms trained on vast libraries of these whole-slide images can analyze the cellular tapestry with unprecedented scale and consistency. AI systems demonstrate proficiency in identifying cancerous regions, including small metastatic deposits that are easily missed by the human eye, while scanning rapidly across large slides. They excel at quantitative tasks that are laborious and inherently variable for humans, such as precisely counting mitotic figures—a key indicator of proliferation—across the entire specimen, or measuring nuanced variations in nuclear size and shape (morphometry).
Beyond replicating or standardizing human tasks, AI is revealing deeper layers of information hidden within standard histology. By analyzing complex spatial relationships between different cell types (tumor cells, immune cells, and stromal cells) and subtle textural features of tissue architecture, AI models are showing potential to directly predict patient prognosis or response to specific therapies from the initial diagnostic slide. Even more strikingly, AI is learning to infer the presence of specific genetic mutations or molecular pathway alterations based purely on the visual patterns in standard HandE-stained tissue – essentially ‘seeing’ the molecular state reflected in cellular morphology. This computational morphology could potentially provide rapid and cost-effective insights that are currently required by separate, slower molecular tests.
This AI-augmented pathology promises not only increased efficiency and consistency but also a richer, more informative diagnostic process. It empowers pathologists by handling demanding quantitative tasks, highlighting areas needing expert attention, and potentially uncovering biological insights previously invisible within the tissue itself. The integration demands careful workflow design and validation. Still, it points towards a future where the pathologist’s interpretive expertise is amplified by powerful computational tools, extracting maximal information from every tissue sample.
Listening for Molecular Whispers: AI and Liquid Biopsy
While imaging and pathology visualize cancer’s physical form, liquid biopsy seeks its fugitive molecular traces – fragments of DNA and other molecules shed by tumor cells into the bloodstream and other bodily fluids. This concept, initially proven in prenatal diagnostics, offers a revolutionary, minimally invasive approach to cancer detection and monitoring. Circulating tumor DNA (ctDNA) carries the mutational and epigenetic signatures of the cancer, providing a potential real-time readout of the disease’s presence and characteristics.
The formidable challenge lies in detecting these scarce ctDNA fragments amidst an overwhelming background of normal cell-free DNA. Often, the cancer-derived signal constitutes less than 0.1% of the total DNA pool. Finding this ‘needle in a haystack’ requires methods of extraordinary sensitivity and specificity. Artificial intelligence is proving indispensable in meeting this challenge. Machine learning algorithms are particularly adept at analyzing the high-dimensional, noisy data generated by deep sequencing of cell-free DNA. They move beyond searching for just one or two specific mutations; instead, they learn to recognize complex, multi-feature signatures characteristic of cancer. These signatures incorporate not only specific sequence alterations but also distinctive patterns in how the DNA is fragmented, chemical modifications like methylation across thousands of genomic regions, and subtle shifts in chromosomal copy numbers. AI integrates these disparate signals to discern the faint but complex molecular fingerprint of cancer with greater reliability than methods relying on more straightforward rules.
This AI-powered analysis is the engine driving the development of multi-cancer early detection (MCED) tests. These blood tests leverage AI to identify pan-cancer signatures, potentially detecting dozens of different cancer types from a single sample, often before conventional screening methods or symptom onset. While still evolving and requiring extensive clinical validation, MCED represents a potential paradigm shift towards proactive, blood-based molecular surveillance. Similarly, AI enhances the use of liquid biopsy for monitoring treatment response, detecting minimal residual disease after surgery, or identifying the emergence of resistance mutations by discerning subtle changes in ctDNA patterns over time. Liquid biopsy, enhanced by artificial intelligence (AI), presents a fundamentally new approach to interacting with cancer at its molecular level.
Tailoring the Search: AI and Precision Screening
Historically, cancer screening has employed a broad-based approach, applying standardized tests to large populations primarily based on age. This ‘one-size-fits-all’ strategy, while beneficial, inevitably screens many who will never develop the disease while missing others at elevated risk. The convergence of AI and genomics enables a more rational, personalized approach: risk-adapted screening.
Central to this is the concept of polygenic risk. While rare, high-impact mutations, such as those in BRCA1/2, confer a significant risk. However, much of the inherited susceptibility to common cancers arises from the combined effect of hundreds or thousands of common genetic variants, each conferring only a small individual risk. Calculating an individual’s polygenic risk score (PRS), which integrates the cumulative impact of numerous variants, requires sophisticated computational methods. AI excels at developing and refining these PRS models, identifying the complex combinations of variants most predictive of disease across vast genomic datasets.
By integrating an individual’s PRS with other factors, such as family history, lifestyle variables, and potentially AI-derived imaging biomarkers like breast density patterns, we can generate a more accurate and personalized estimate of their cancer risk. This enables tailored screening strategies: high-risk individuals may receive earlier, more frequent, or more sensitive screening tests (e.g., MRI instead of just mammography), while low-risk individuals may safely undergo less intensive screening, thereby optimizing resource allocation and minimizing the harms of over-screening. While implementing risk-adapted screening requires careful consideration of ethical issues and ensuring equitable access, AI provides the analytical engine needed to make this personalized vision a practical reality.
Convergence: Towards Integrated, Dynamic Diagnosis
The most profound transformation arises not from AI enhancing individual modalities in isolation, but from its ability to synthesize information across them. Cancer diagnosis is inherently multi-modal; clinicians integrate patient history, physical findings, lab results, imaging reports, pathology assessments, and molecular data. Multi-modal AI aims to replicate and potentially enhance this complex integration computationally.
Imagine an AI system analyzing a suspicious lung nodule on a CT scan not just based on its visual features, but simultaneously considering the patient’s polygenic risk score for lung cancer, subtle methylation patterns detected in a concurrent liquid biopsy, and quantitative features extracted from prior pathology reports, if available. By learning the complex correlations between these diverse data streams, such systems could potentially achieve diagnostic and prognostic accuracy that exceeds that of any single modality alone.
This suggests a future of more dynamic and continuous health assessment. Rather than relying solely on episodic snapshots from scans or biopsies, AI could integrate longitudinal data from multiple sources – perhaps including wearables or patient-reported outcomes – to maintain an evolving picture of an individual’s risk and health status, enabling intervention at the earliest possible sign of change. This represents a fundamental shift from reacting to diagnosed disease towards proactively managing health trajectories.
Conclusion: Seeing Cancer Anew
The integration of artificial intelligence into cancer detection is not merely an incremental improvement; it is fostering a revolution in medical perception. We are developing tools that extend beyond the limits of human senses and cognitive capacity, enabling us to detect the presence and nature of cancer with unprecedented sensitivity and depth. From interpreting the subtle textures within radiological images, to quantifying the complex cellular interactions on a pathology slide, to decoding the faint molecular whispers in the bloodstream, AI provides powerful new lenses.
This emerging paradigm, built on a synergistic partnership between human expertise and machine intelligence, promises to reshape the landscape of oncology. It facilitates earlier detection, potentially intercepting cancers when they are most treatable. It enables the more precise characterization of individual tumors, guiding the development of more effective and personalized therapies. It offers the potential for more consistent, equitable, and efficient diagnostic processes. While the journey from potential to widespread, equitable practice requires navigating significant technical, regulatory, and societal challenges, the fundamental shift in our ability to ‘see’ cancer is undeniable. We are moving towards a future where this ancient adversary can be met not with fearful uncertainty, but with the clarity and foresight afforded by these powerful new eyes.”
More posts featuring Douglas Flora.
-
Challenging the Status Quo in Colorectal Cancer 2024
December 6-8, 2024
-
ESMO 2024 Congress
September 13-17, 2024
-
ASCO Annual Meeting
May 30 - June 4, 2024
-
Yvonne Award 2024
May 31, 2024
-
OncoThon 2024, Online
Feb. 15, 2024
-
Global Summit on War & Cancer 2023, Online
Dec. 14-16, 2023